class: center, top, title-slide .title[ #
Exponential Growth: Part I
] .subtitle[ ##
EFB 370: Population Ecology
] .author[ ###
Dr. Elie Gurarie
] .date[ ###
February 10, 2025
] --- <!-- https://bookdown.org/yihui/rmarkdown/xaringan-format.html --> .pull-left[ ## Goals ### (concepts / buzzwords / jargon) - The Population Equation - Closed vs. Open Population - Unconstrained (density independent) Growth - Geometric Growth = Exponential Growth - Discrete Time vs. Continuous Time Models - Difference Equations vs. Differential Equations - Population growth parameters: - continuous / discrete / per capita / per unit time - Amazing properties of exponential and log. ] .pull-right[ ## *Suggested* reading - Gotelli: Chapter 1 (first half) - Neal: Chapter 4 ] --- class:Large ## Meet ... *Enhydra lutris* <img src="images/seaotterrocks.jpg" width = 600 style="float: left; padding: 5px 40px 5px 5px;" align = "left"/> The largest ... -- .center[**Mustelid**] The smallest ... -- .center[**Marine mammal**] By far the furriest! Guess how may hairs / sq. inch ? (*Hint, humans are born with ~100,000 TOTAL.* ) -- .center[**1,000,000** (ish!)] --- ## Sea otters: Range .pull-left-60[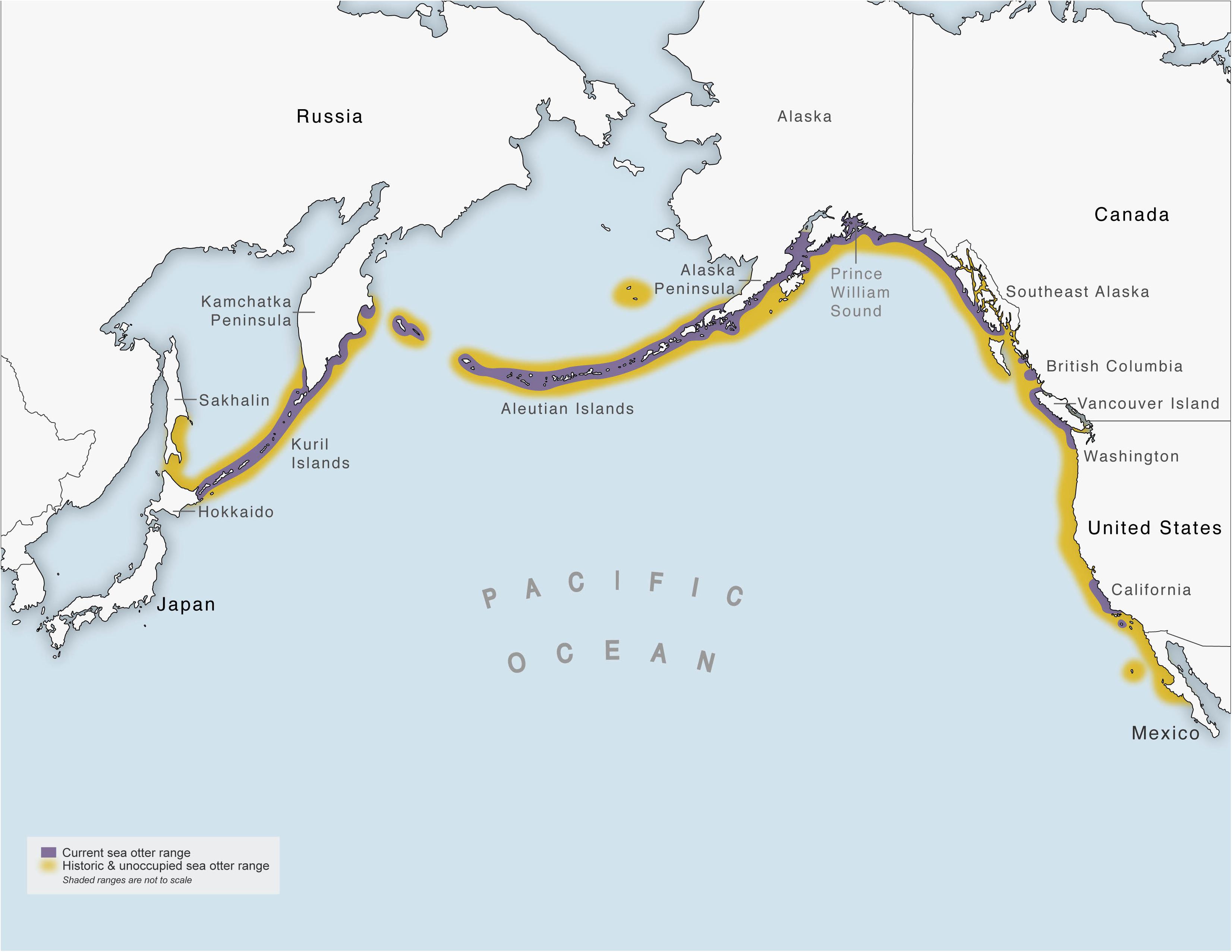] .pull-right-40.Large[*Littorally* the entire North Pacific] --- ## Sea otters: Keystone Species 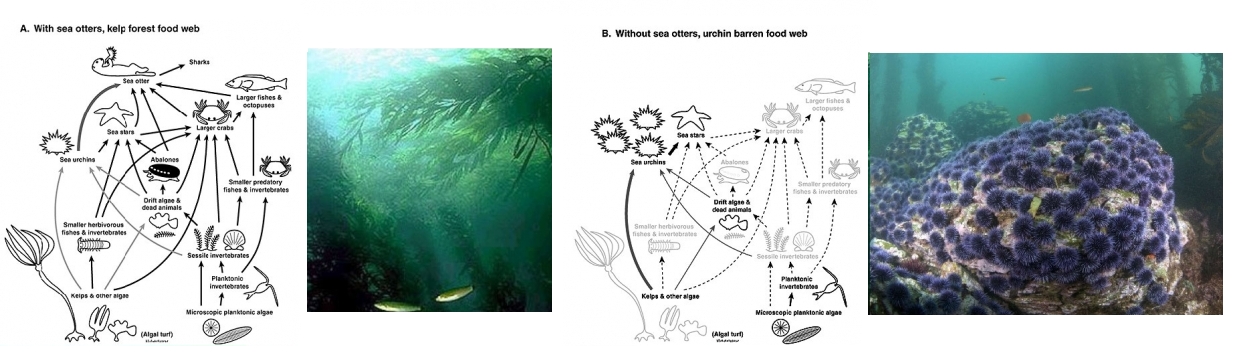 (Estes et al. 1974) --- .pull-left-50[ ## Sea otters: Furriness > Cuteness 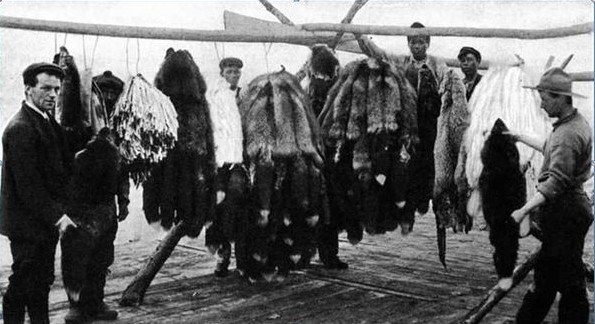 ] .pull-right-30[ 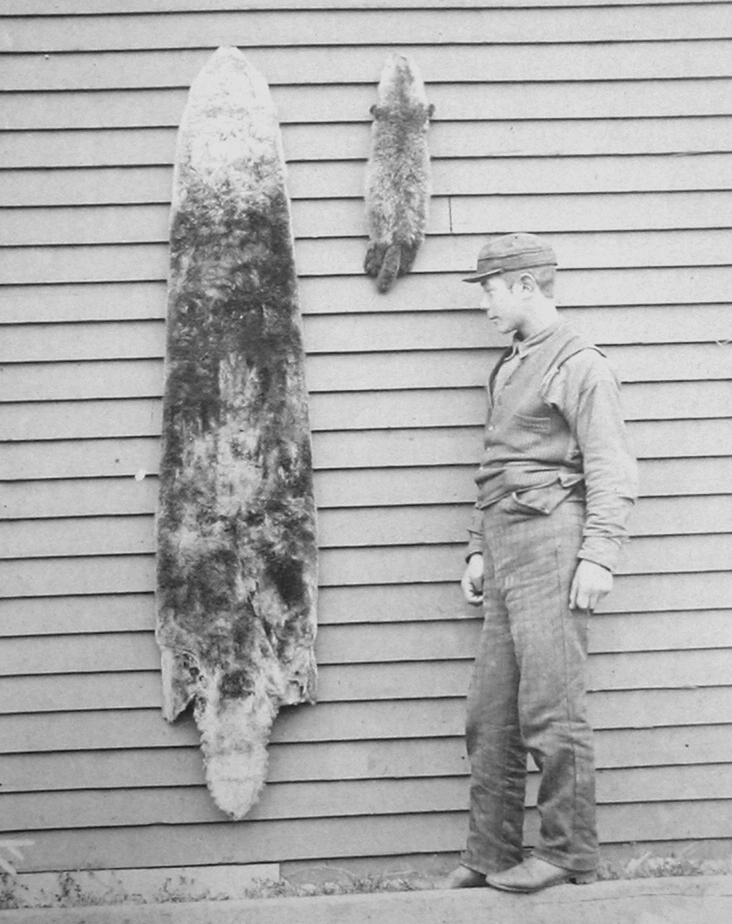 ] .pull-left[ - Fur trade (Russian -> British -> American) leads to near **extirpation** across the entire range. - **> 300,000 in 1740 ... < 2,000 in 1900**. - Displacement and indenturing of Indigeneous fishermen (esp. Aleut) ] .pull-right[ > .footnotesize[*... the rush for the otters’ “soft gold” was a predictable boom and bust ... a cautionary example of unsustainable resource use, and a socioeconomic driver of Western — mainly American — involvement in the Pacific region,* (Loshbaugh 2021)] ] --- .pull-left[ ## Sea otters: Not totemic .box-grey[ ... a sea otter hunter who was “lazy, spiteful, malicious or disregarded the teaching of the elders” would find his prey “cavorting around his baidarka ... teas[ing] and splash[ing] him with water.” *(Innokentiy Venianimov, 1840)* ] 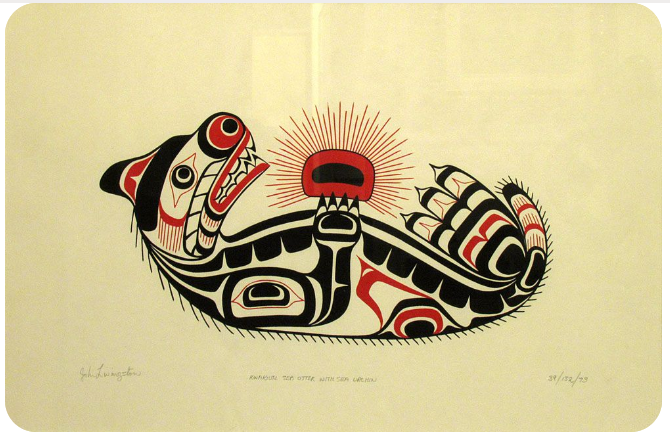 .center[*art by John Livingston*] ] .pull-right[ ### ... but culturally significant <iframe src="https://www.youtube.com/embed/ZyYH4KBHKU4?controls=0" width = "400" height = "240"> </iframe> .small[See: https://www.youtube.com/watch?v=ZyYH4KBHKU4] .scriptsize[ .pull-left[ | culture | name | |---|---| | [Ainu](https://en.wikipedia.org/wiki/Ainu_people) | Esaman | | [Koryak](https://en.wikipedia.org/wiki/Koryaks) | Kolakha | | [Aleut](https://en.wikipedia.org/wiki/Aleut) | Chngatux | | [Alutiiq](https://en.wikipedia.org/wiki/Alutiiq) | Arhnaq | ] .pull-right[ | culture | name | |---|---| | [Tlingit](https://en.wikipedia.org/wiki/Tlingit) | Yáxwchʼ | | [Haida](https://en.wikipedia.org/wiki/Haida) | Ku | | [Nuu chah nulth](https://en.wikipedia.org/wiki/Nuu-chah-nulth) | Кwak̕aƛ | | [Siletz](https://en.wikipedia.org/wiki/Siletz) | Elakha | ] ] ] --- ## Sea otter reintroduction: Pacific NW Remnant populations from Aleutian Islands ... released in OR, WA, BC and SE-AK 1969 – 1972. .center[ <img src="images/introduction.jpg" alt="Reintroduction trade" height="300"/> <img src="images/introduction2.jpg" alt="Reintroduction trade" height="300"/> ] --- ## Sea otter reintroduction: Washington State ... .pull-left[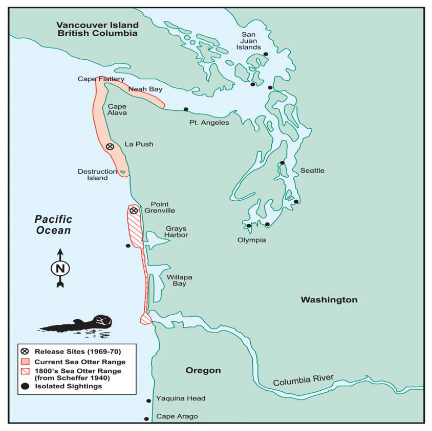] .pull-right[ .pull-left[1970: **59 otters**] .flush-right[2010's: **over 1000**] 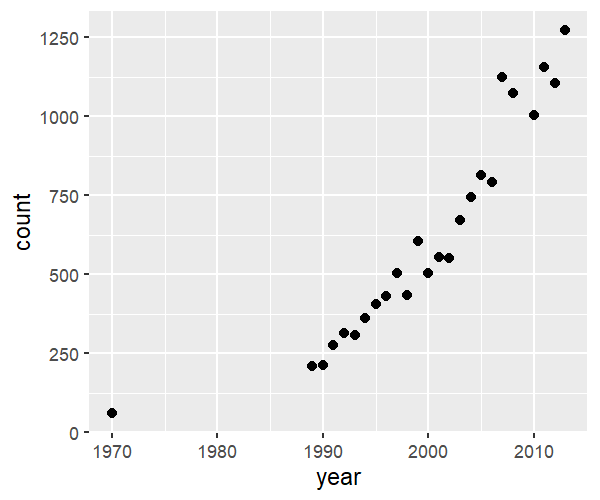 ] --- ## Sea otter reintroduction: Washington State ... .pull-left[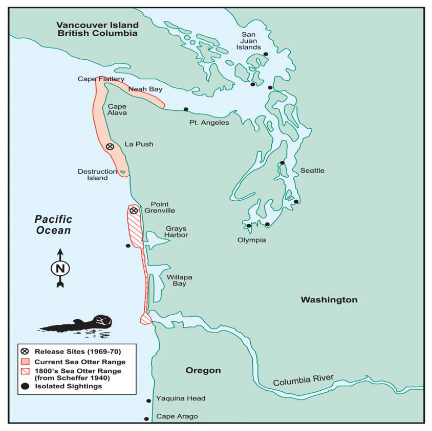] .pull-right[ .pull-left[1970: **59 otters**] .flush-right[2010's: **over 1000**] 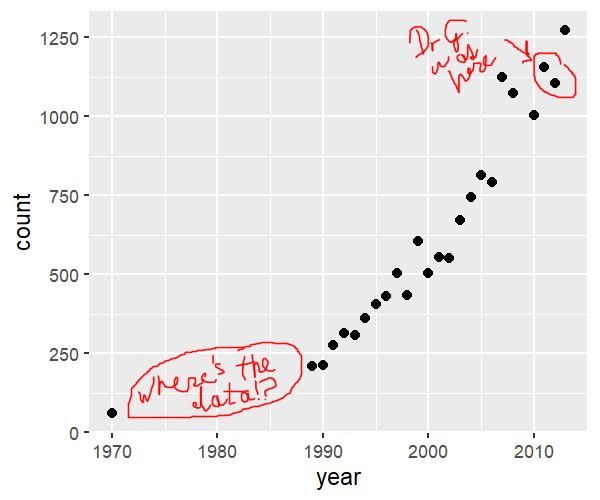 ] .center.Large[***Success!***] --- ## Population ecology is all about ... `$$\huge N$$` but where? when? -- ## Here! Now! .. `$$\huge N_t$$` but how many were there? --- ## That many, then `\(\Delta t\)` ago! `$$\Large N_t = N_{t - \Delta t} + \Delta N$$` slight rearrangement: `$$\Large N_{t+1} = N_t + \Delta N$$` For now, `\(\Delta t = 1\)`, i.e. it's the discrete unit that we measure population change. VERY TYPICALLY - whether because of biology or field seasons: `$$\Delta t = 1\,\, \textrm{year}$$`. --- ## How does population change? .pull-left-30[ ### **B**irth ### **D**eath ### **I**mmigration ### **E**migration ] .pull-right-70[ `$$\Large N_{t+1} = N_t + (B - D) + (I - E)$$` .center[This is the **Fundamental population equation**.] 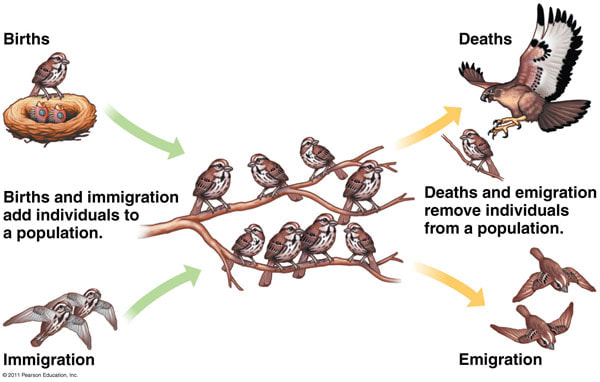 ] --- ## Assumption 1: **Closed Population** `$$\Large N_{t+1} = N_t + B - D$$` .pull-left-30[ ### **B**irth ### **D**eath ### .red[**I**mmigration] ### .red[**E**migration] ] .pull-right-70[ 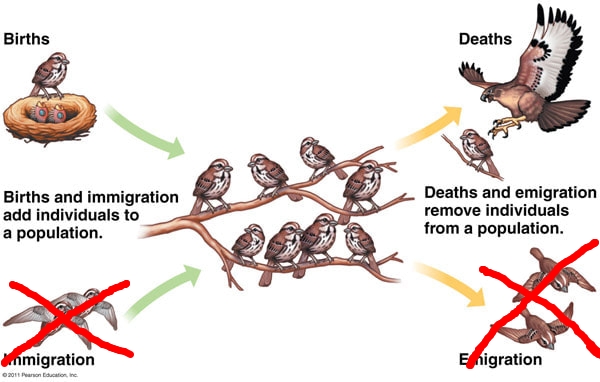 ] --- ## Assumption 1: no one's getting on or off the bus `$$\Large N_{t+1} = N_t + B - D$$` ### **B**irth ### **D**eath ### ~~.red[**I**mmigration]~~ ### ~~.red[**E**migration]~~ .center[This is a .darkblue[**closed**] (vs. **open**) .darkblue[**population**] ...] --- ## Assumption 2: the important one The number of *Births* and *Deaths* is proportional to *N*. `$$\Large N_{t+1} = N_t + bN_t - dN_t$$` *b* and *d* are number of births and deaths *per individual*. What does that mean? -- .pull-left[ .box-yellow[**Births** - Every female gives birth to the same number of offspring? - Every female has the same *probability* of giving birth to a single offspring? - Every female has the same *probability* of giving birth to the same *distribution* of offspring?] ] .pull-right[ .box-green[ **Deaths** - A fixed proportion of all individuals dies? - Every individual has the same *probability* of dying? - the *distribution* of *probabilities* of dying is constant? ]] .center.large[**These are all subtly & importantly different!**] --- ## Some math .... Define `$$r_0 = b - d$$` `\(r_0\)`: **discrete growth factor**, `$$N_{t+1} = N_t + r_0 N_t$$` `$$N_{t+1} = (1 + r_0) N_t$$` `$$N_{t+1} = \lambda N_t$$` `\(\lambda\)` is .blue[**rate of growth (or decrease)**] - If `\(d > b\)`, `\(\lambda < 1\)`. - If `\(b > d\)`, `\(\lambda > 1\)`. --- ## Cranking this forward `$$N_{t+1} = \lambda(N_t)$$` `$$N_{t+2} = \lambda(N_{t+1}) = \lambda^2 N_t$$` `$$N_{t+3} = \lambda^3 N_t$$` Solution: `$$\large N_{t+y} = \lambda^y N_t$$` or `$$\huge N_t = \lambda^t N_0$$` .center[***Geometric*** (same as ***Exponential***) growth.] --- ## Some examples 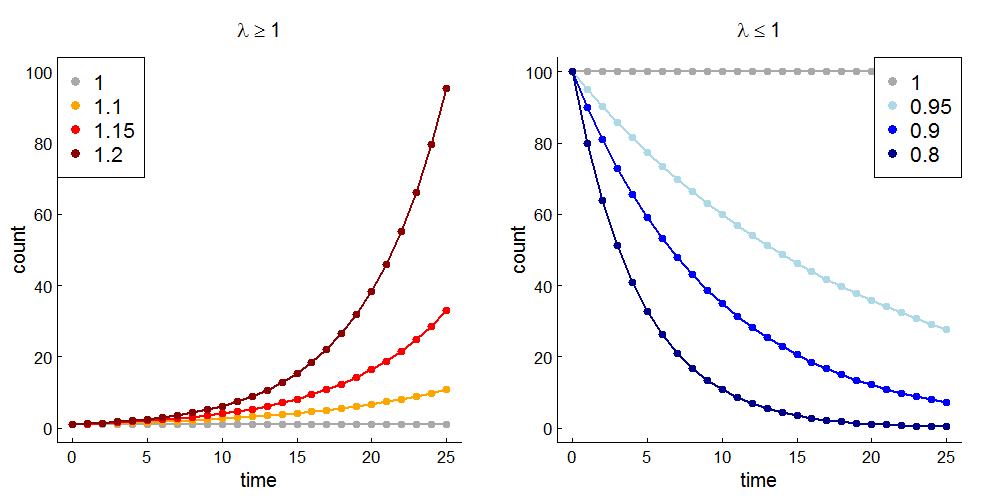<!-- --> --- ## How fast is exponential/geometric growth? .pull-left-60[ 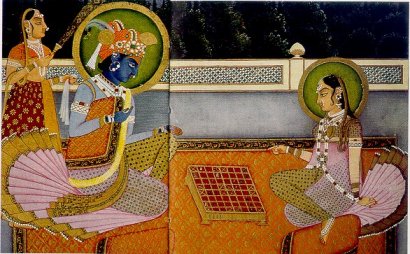 Legend says the inventor of chess (in India) so delighted the raja, he was offered anything he wanted. Inventor said, not much. One grain of rice on one square, 2 on the next, 4 on the next and so on till the board is filled. ] -- .pull-right-40[ 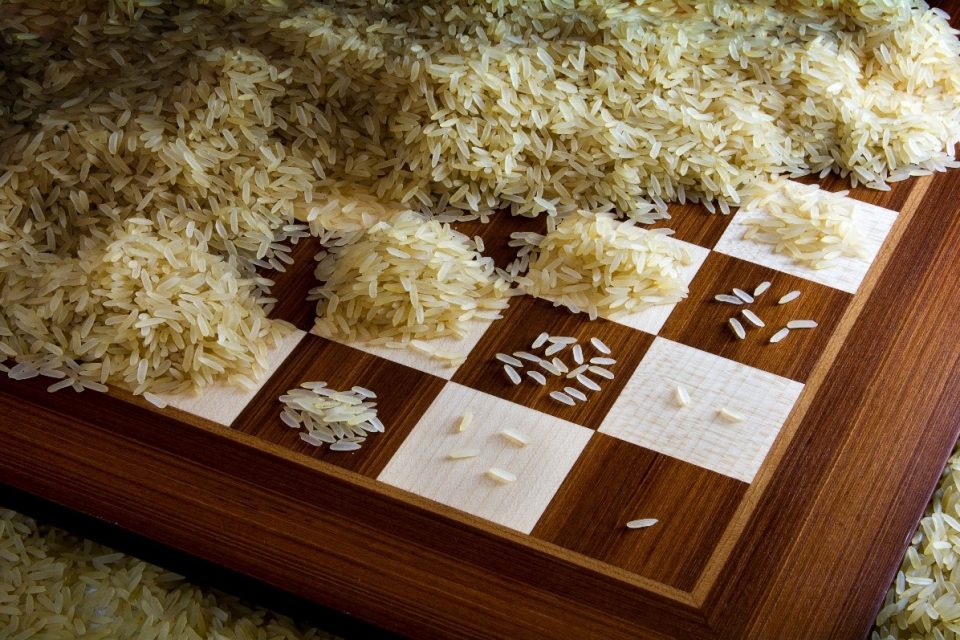 Not only is there not enough rice in India to fill such a chessboard, **there are not enough atoms on earth**. ] --- ## Note what the log-scale does 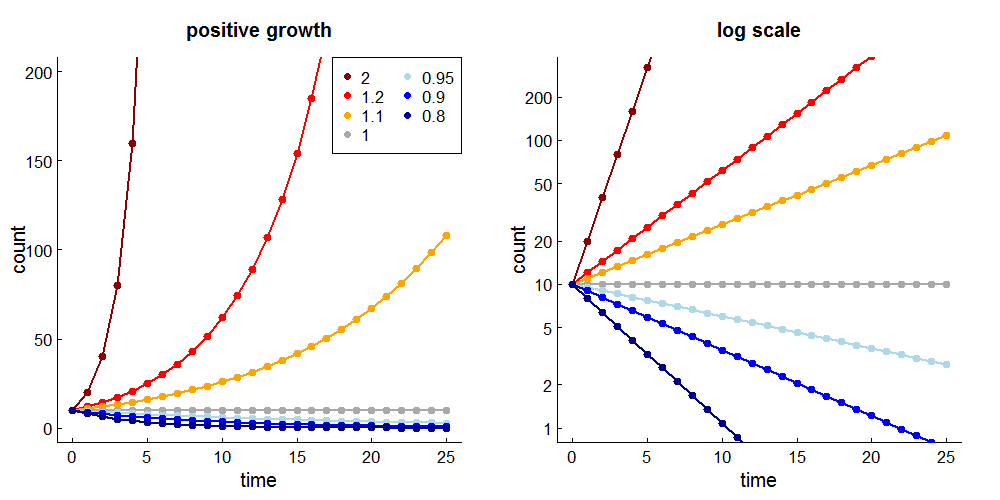<!-- --> --- ## Estimating some rates ... discrete .pull-left-30[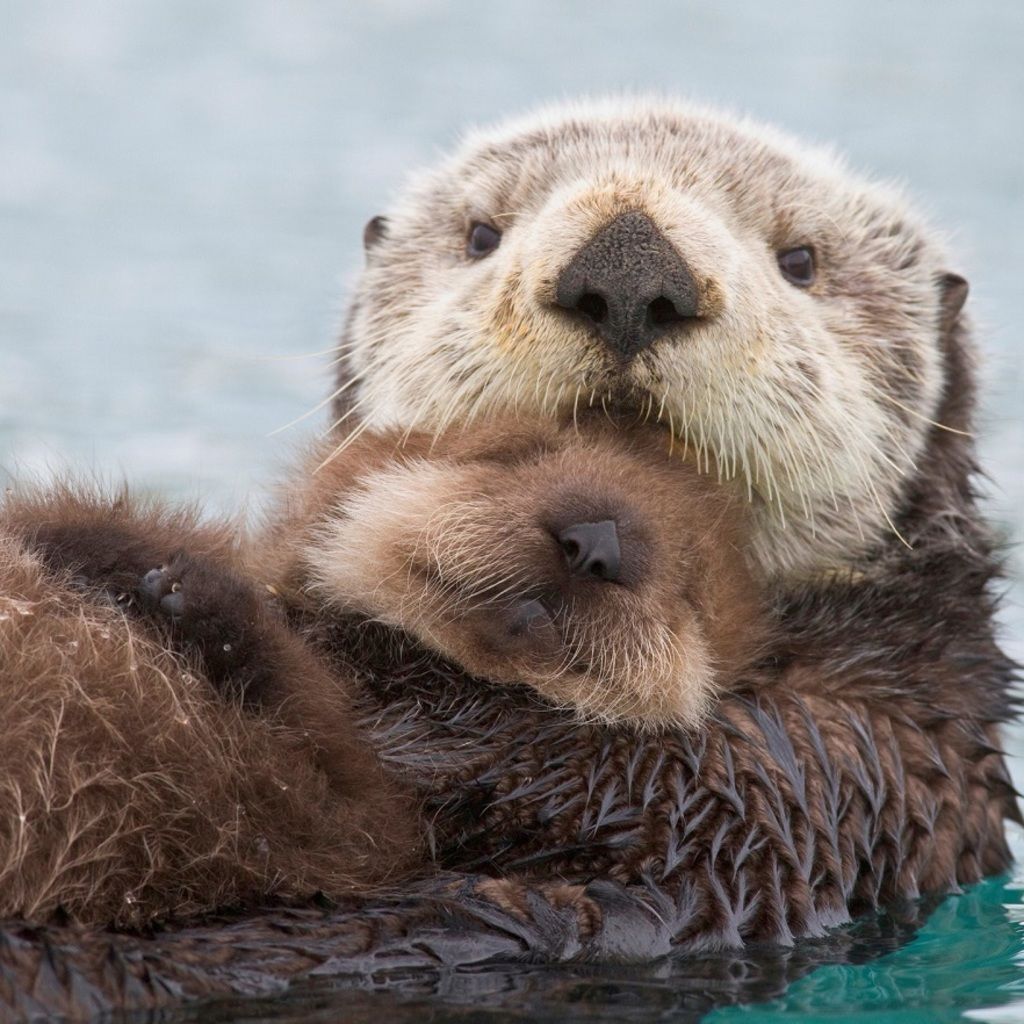] .pull-right-70[ The amazing thing is, if you have an equation "solved", you only need 2 points on the curve to compute. Let's use the discrete equation: `$$N_{t+y} = \lambda^y N_t$$` `$$1000 = 60 \times \lambda^{40}$$` `$$16.7 = \lambda^{40}$$` `$$2.81 = 40 \times \log (\lambda)$$` `$$0.07025 = \log (\lambda)$$` `$$\lambda = \exp(0.07025) = 1.0728$$` i.e. population increase about `$$7.28\%/year$$`. ] --- ## Washington sea otter fit to data (7.025% discrete growth) .pull-left-70[  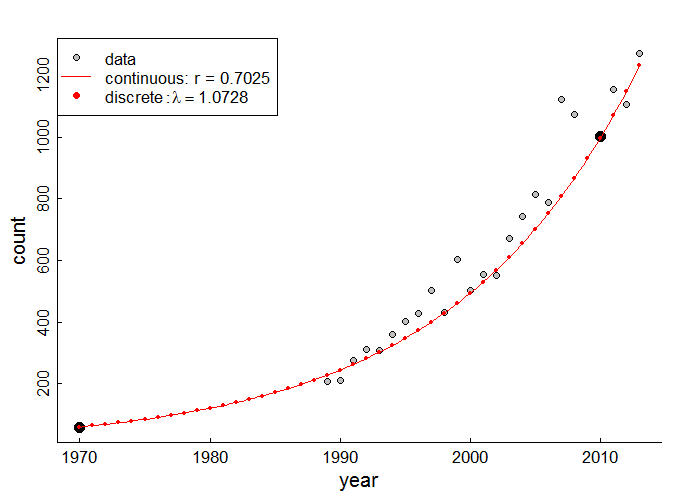<!-- --> ] .pull-right-30[ ### This is an EXCELLENT fit but why isn't it *perfect*? .darkgreen[What are some potential sources of **variation**?] ] --- ## Discrete Model to Continuous Model .pull-left[ Let's do some trickery, starting with: `$$N_{t+1} = (1 + r_0) \, N_t$$` `$$N_{t+1} - N_t = r_0 N_t$$` `$$N_{t+\Delta t} - N_t = r_{\Delta t} N_t$$` `$$\lim_{\Delta t \to 0} {\Delta N \over \Delta t} = \lim_{\Delta t \to 0} {r_{\Delta t} \over \Delta t} N$$` ] .pull-right[ Magically define: `$${r_\Delta \over \Delta t} = r$$` and rewrite `\(\Delta\)` as `\(d\)`: .blue[$$\large {dN \over dt} = r N$$] > .darkblue.large[This is a ***differential*** (vs. difference) equation] ] --- .large.green[**Solve this:** `$$\large {dN \over dt} = r N$$`] where: `$$N(t = 0) = N_0$$` .pull-left[ .small[Integration is fun! (*but you don't need to know how to do this*)] `$$\begin{align} {1\over N} dN &= r dt \\ \int_{t' = t_0}^t {1 \over N(t)} dN &= \int_{t' = t_0}^t r dt \\ \log(N) &= rt + C_0 \\ N &= e^{rt + C_0} \\ \end{align}$$` ] .pull-right[ .large.red[**Solution:** `$$\large N(t) = N_0 e^{rt}$$`] > **Incredibly Interesting And Important Fact:** >The exponential function is the ONLY function whose derivative is proportional to itself. ] --- ## Some examples 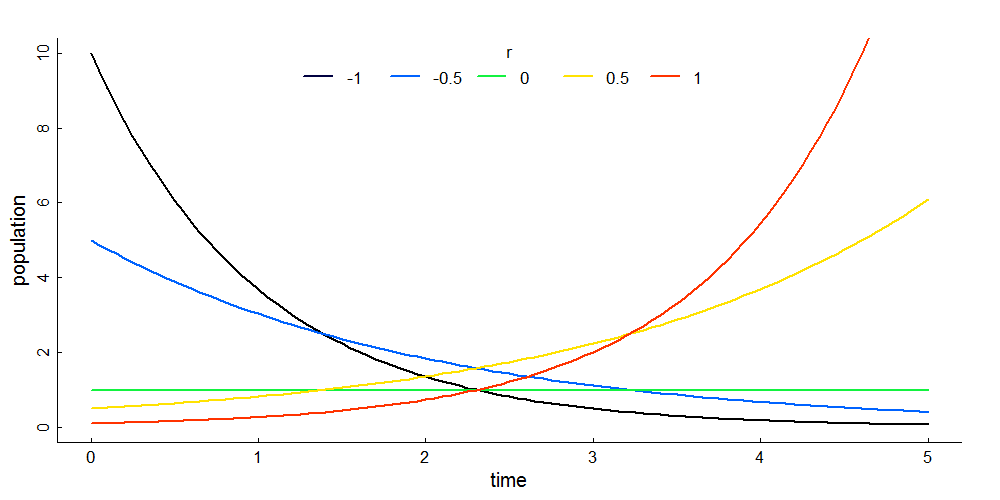<!-- --> --- .pull-left[ ## Note crazy fast growth 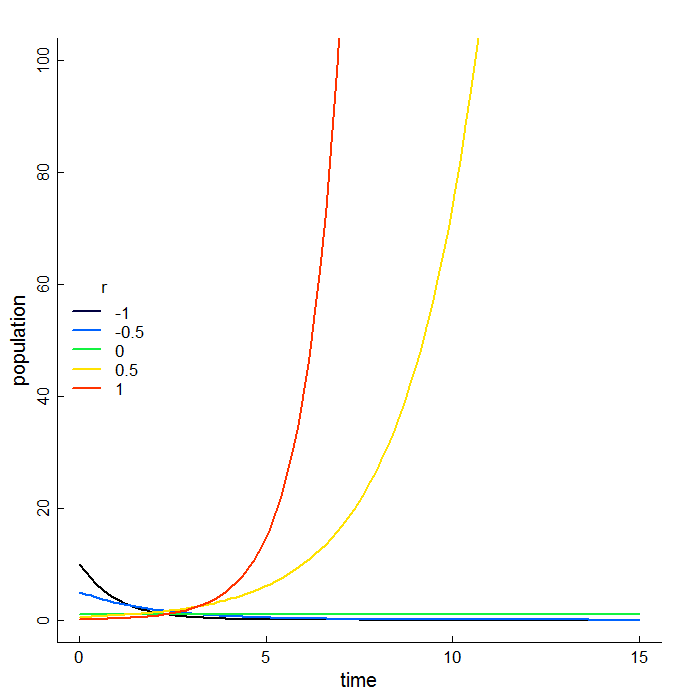<!-- --> ] .pull-right[ ## and how straight on a log-scale 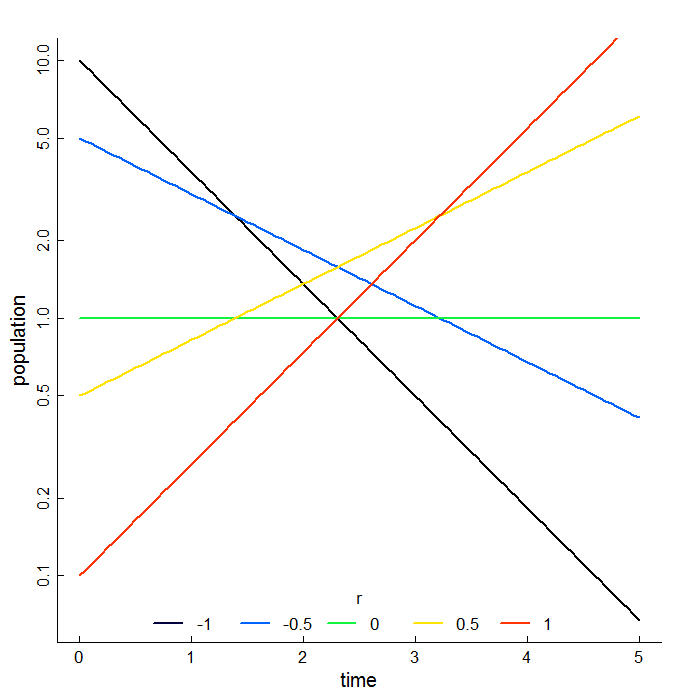<!-- --> ] --- ## Discrete vs. Continuous Modeling .pull-left[ **Difference equations** `$$N_{t+\Delta t} - N_t = \lambda_{\Delta t} N_t$$` think of *absolute change* **Pros:** - Reflects (often) biological reproduction patterns, practical sampling schedule (esp. annual) - Intuitive **Cons:** - Depends on discretization timescale - Surprisingly difficult to analyze ] .pull-right[ **Differential equations** `$${dN \over dt} = r N$$` think of *rates* (change/time). **Pros** - Easier "elegant" mathematical analysis - Scales nicely **Cons** - Unbiological - Unintuitive ] --- ## Estimating some rates ... continuous .pull-left-30[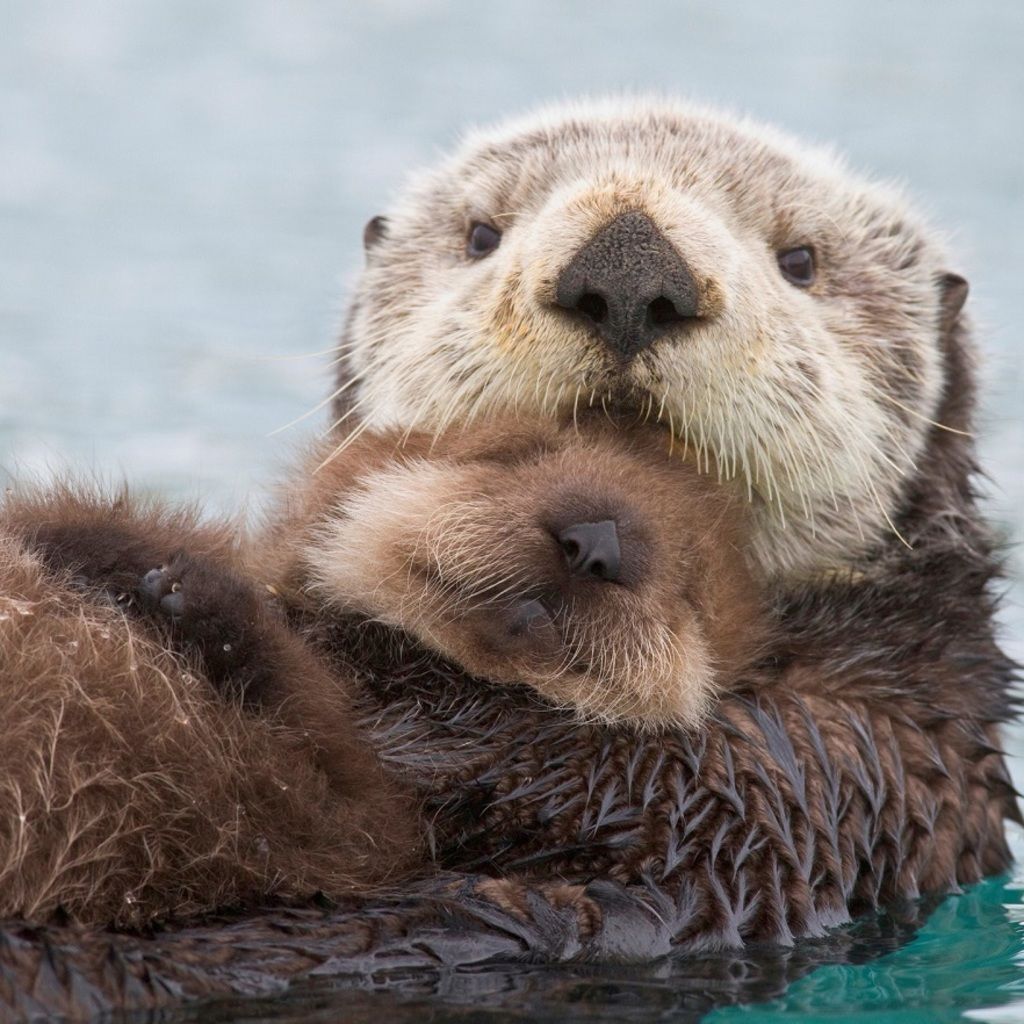] .pull-right-70[ Let's use the continuous equation: `$$\begin{align} 1000 &= 60 \times e^{40\,r}\\ 16.7 &= e^{40 \, r}\\ 2.81 &= 40 \times r\\ r &= 0.07025 \end{align}$$` .large[*Intrinsic growth rate* = 0.07025.] ] --- ## Washington sea otter fit to data .pull-left[ 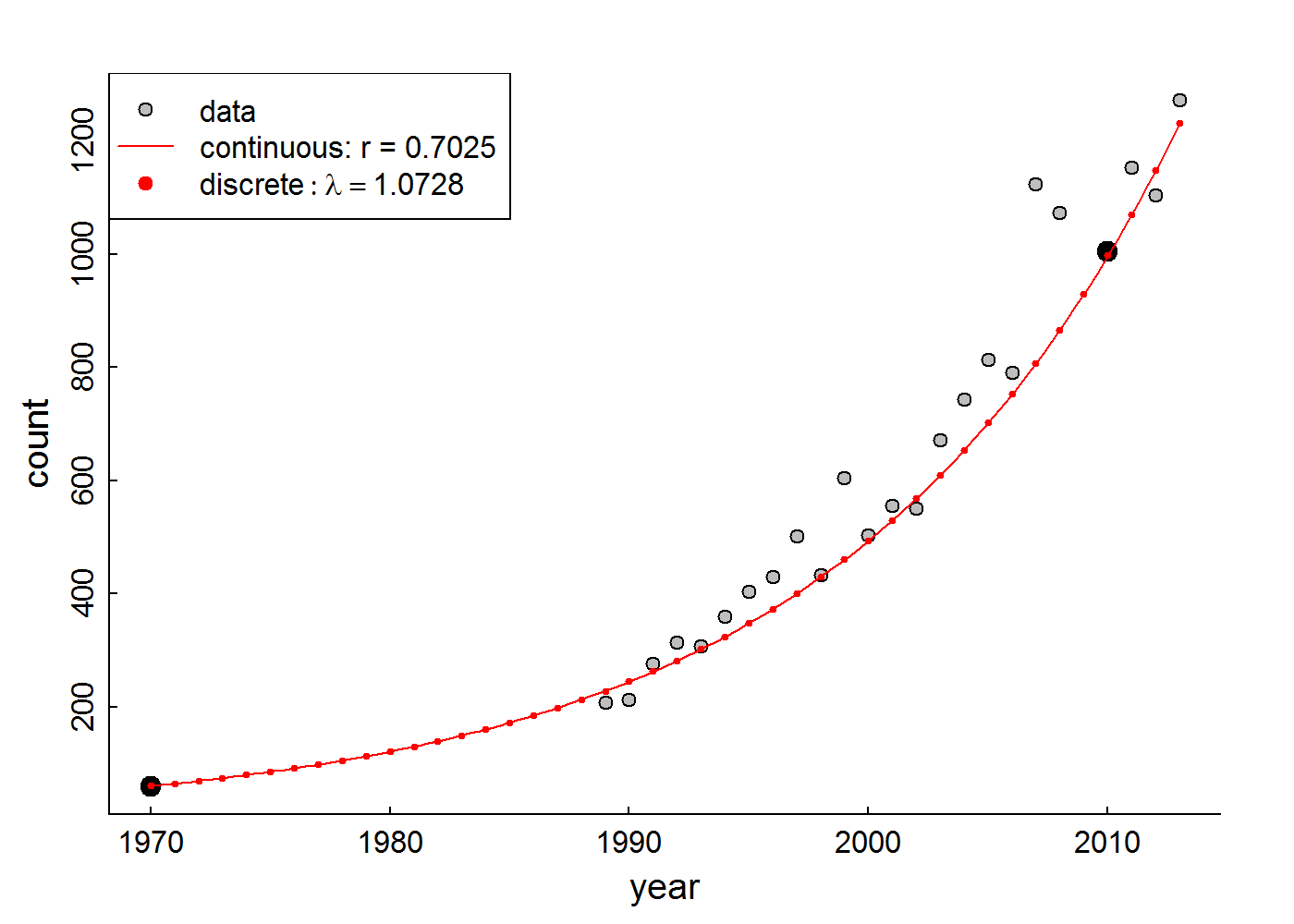<!-- --> ] .pull-right[ Note: dots for **discrete**, lines for **continuous** ... .green[*but really there is NO difference in the two curves*] ] --- ## Estimating some rates ... discrete .pull-left-30[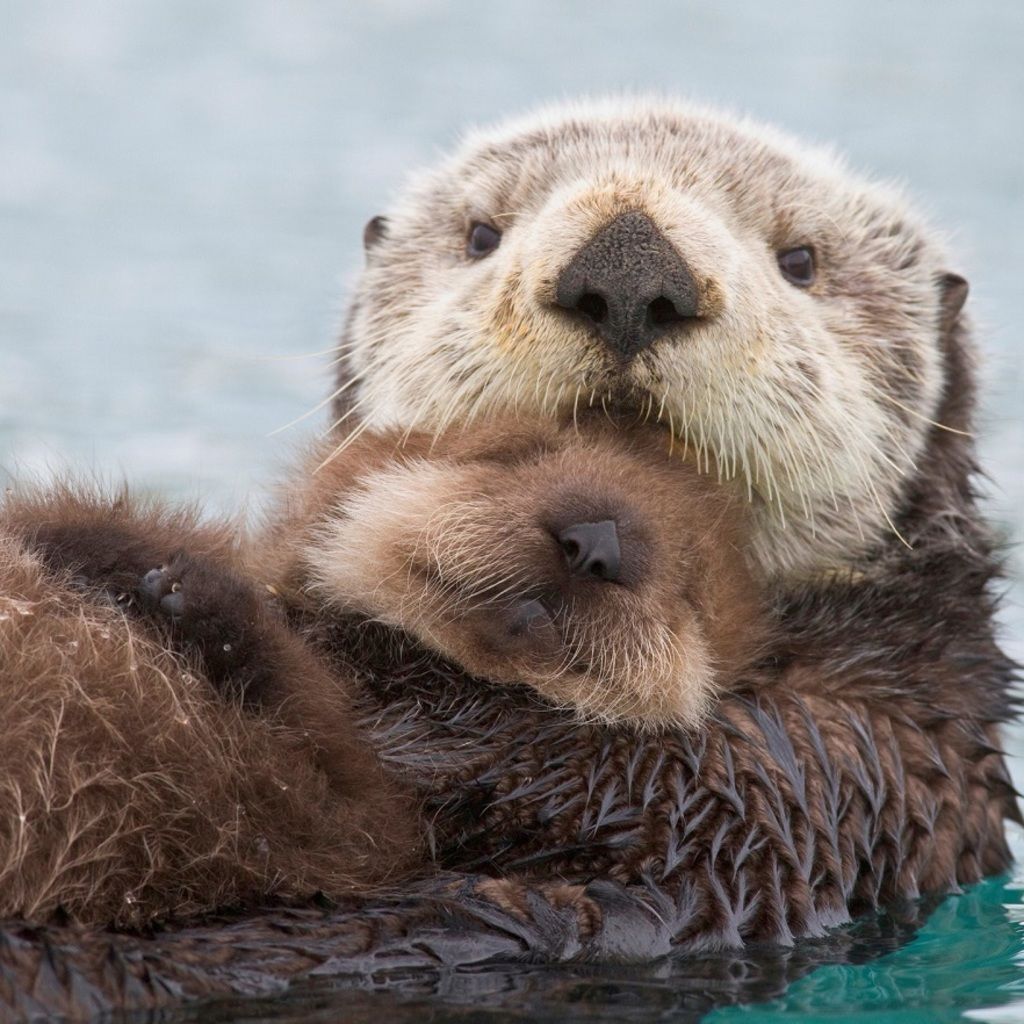] .pull-right-70[ The amazing thing is, if you have an equation "solved", you only need 2 points on the curve to compute. Let's use the discrete equation: `$$N_{t+y} = \lambda^y N_t$$` `$$1000 = 60 \times \lambda^{40}$$` `$$16.7 = \lambda^{40}$$` `$$2.81 = 40 \times \log (\lambda)$$` `$$0.07025 = \log (\lambda)$$` `$$\lambda = \exp(0.07025) = 1.0728$$` i.e. population increase about `$$7.28\%/year$$`. ] --- ## How long does it take for a population to double? .center.large[ solve for doubling time `\(t_d\)` ] .pull-left.large[ **Continuous:** `$$2N = N e^{rt_d}$$` `$$\log(2) = rt_d$$` `$$t_d = \log(2)/r$$` ] .pull-right.large[**Discrete:** `$$2N = N \lambda^{t_d}$$` `$$\log(2) = \log(\lambda) t_d$$` `$$t_d = \log(2)/\log(\lambda)$$` ] -- .center.large[ > Sea otter population doubles in ~10 years. ] --- ## Some sea otter references .pull-left[ - J. A. Estes, J. F. Palmisano. 1974. Sea otters: Their role in structuring nearshore communities. Science 185, 1058–1060. ([link](https://www.science.org/doi/10.1126/science.185.4156.1058)) - Loshbaugh S. 2021. Sea Otters and the Maritime Fur Trade. In: Davis R.W., Pagano A.M. (eds) *Ethology and Behavioral Ecology of Sea Otters and Polar Bears. Ethology and Behavioral Ecology of Marine Mammals.* ([link](https://link.springer.com/chapter/10.1007/978-3-030-66796-2_10)) - Gilkinson, A.K., Pearson, H.C., Weltz, F. and Davis, R.W., 2007. Photo‐identification of sea otters using nose scars. *The Journal of Wildlife Management*, 71(6), pp.2045-2051. ([link](https://doi.org/10.2193/2006-410)) - Veltre, D.W. *Unangax̂: Coastal People of Far Southwestern Alaska*. ([link](https://www.apiai.org/departments/cultural-heritage-department/culture-history/history/)) ] .pull-right[ 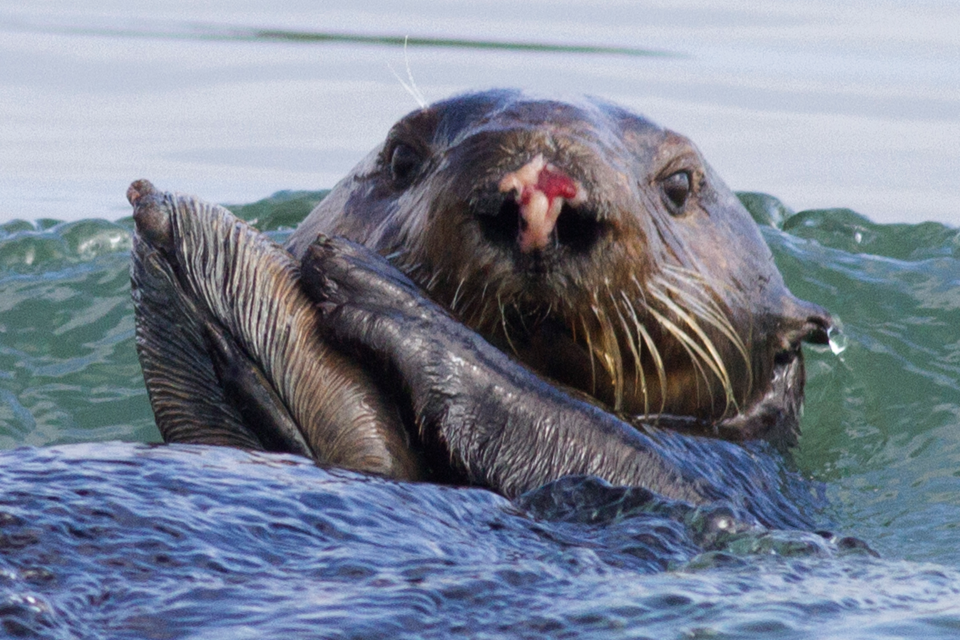 If you want to disabuse yourself of "sea otters are cute" - read about why the females have nose scars. ]