class: center, top, title-slide .title[ #
Limits on Population Growth: Part II
] .subtitle[ ##
.white[EFB 370: Population Ecology]
] .author[ ###
Dr. Elie Gurarie
] .date[ ### February 28, 2025 ] --- class:large <!-- https://bookdown.org/yihui/rmarkdown/xaringan-format.html --> class: inverse # Topics .Large[ - Wolf carrying capacity example & experiment - Delayed Density Dependence - Discrete Logistic Growth - Delayed Discrete Density Dependence ] --- .pull-left-50[ ## Basics of Wolf population dynamics - Dispersal into new area, mainly wolf mating pairs. - Highly territorial! - Wolves produce up to 4 pups per litter that survive - If there are no neighbors, wolves will disperse to found new packs - Pack with 8 adults or 2 adults, still produces (about) 4 pups per litter - If there are lots of neighbors, packs become larger (more individuals) in smaller territories. <img src='images/WI_WolfTerritorySize.png' width='80%'/> ] .pull-right-50[ **Expansion of Wisconsin Wolves, 1970's to 2000's** 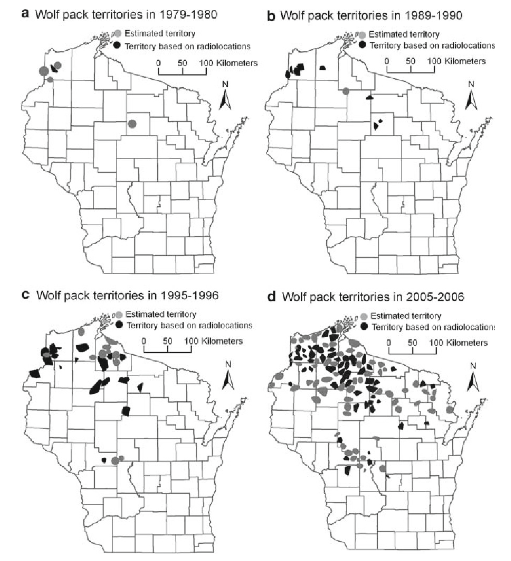 ] --- ## Human-wolf experiment .pull-left-60[ ### basics of model - 8 possible territories - 1 initial dispersing wolf (female) ### each season ... - One female / pack gives birth to 2 offspring - Offspring can choose whether to disperse or not - about 1/4 of all wolves die each year ] .pull-right-40[ 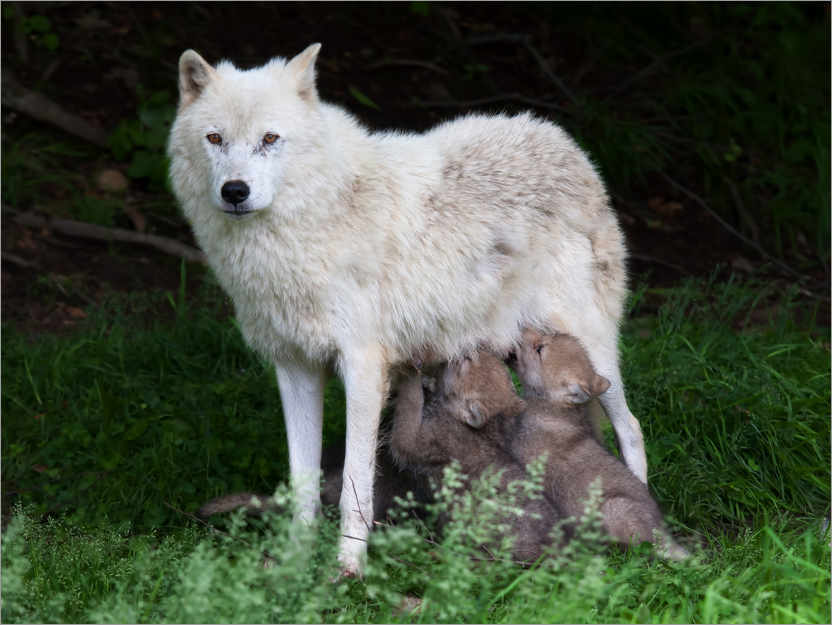 ] --- .pull-left-60[ ### Summary Concepts Growth of natural populations is always *eventually* limited When population rates (*b*, *d*, also *i*, *e*) depend on the **total population** or **density** (***N***), this is called: .darkred[***Density Dependence***]. The maximum growth rate ( `\(r_0 = max (b-d)\)`) is called the .darkred[**intrinsic growth rate**]. In density dependent growth, the .darkred[actual growth rate (***r***)] falls with higher density until it is 0. The point where that happens is the .darkred[***Carrying Capacity*** (**K**)]. If ***N*** ever exceeds ***K***, the growth becomes negative. .darkred[***Logistic growth***] is a specific kind of .darkred[Density Dependent] growth where the relationship between ***r*** and ***N*** is .darkred[**linear**]. The formula is: `$$r = r_0 (1 - N/K)$$` which leads to the following differential equation: `$${dN \over dt} = r_0 N \left( 1 - {N \over K}\right)$$` ] --- ## *Delayed* Density Dependence *Sometimes* (or often?) the *density dependent response* kicks in with a *time lag*. .pull-left[ **Logistic Growth** `$$\Large {dN \over dt} = r N_t \left(1 - {N_t \over K}\right)$$` ] .pull-right[ **Logistic Growth** .red[**with delay**] `$$\Large {dN \over dt} = r N_t \left(1 - {N_{t - \color{red}{\tau}} \over K}\right)$$` ] > Can you think of examples? --- ## simulation (sadly - not mine) .center[https://demonstrations.wolfram.com/DelayLogisticEquation/] --- ## Delayed Logistic Growth .pull-left-30[ The *greater* the delay (`\(\tau\)`), the *greater* the **amplitude** of oscillation. Oscillations are defined by: - **amplitude** - **period** .green[**mathemagically:** the period is always `\(= 4\tau\)` ] If `$$r \times \tau > 1.57$$` the oscillation attains a **stable limit cycle** ] .pull-right-70[ 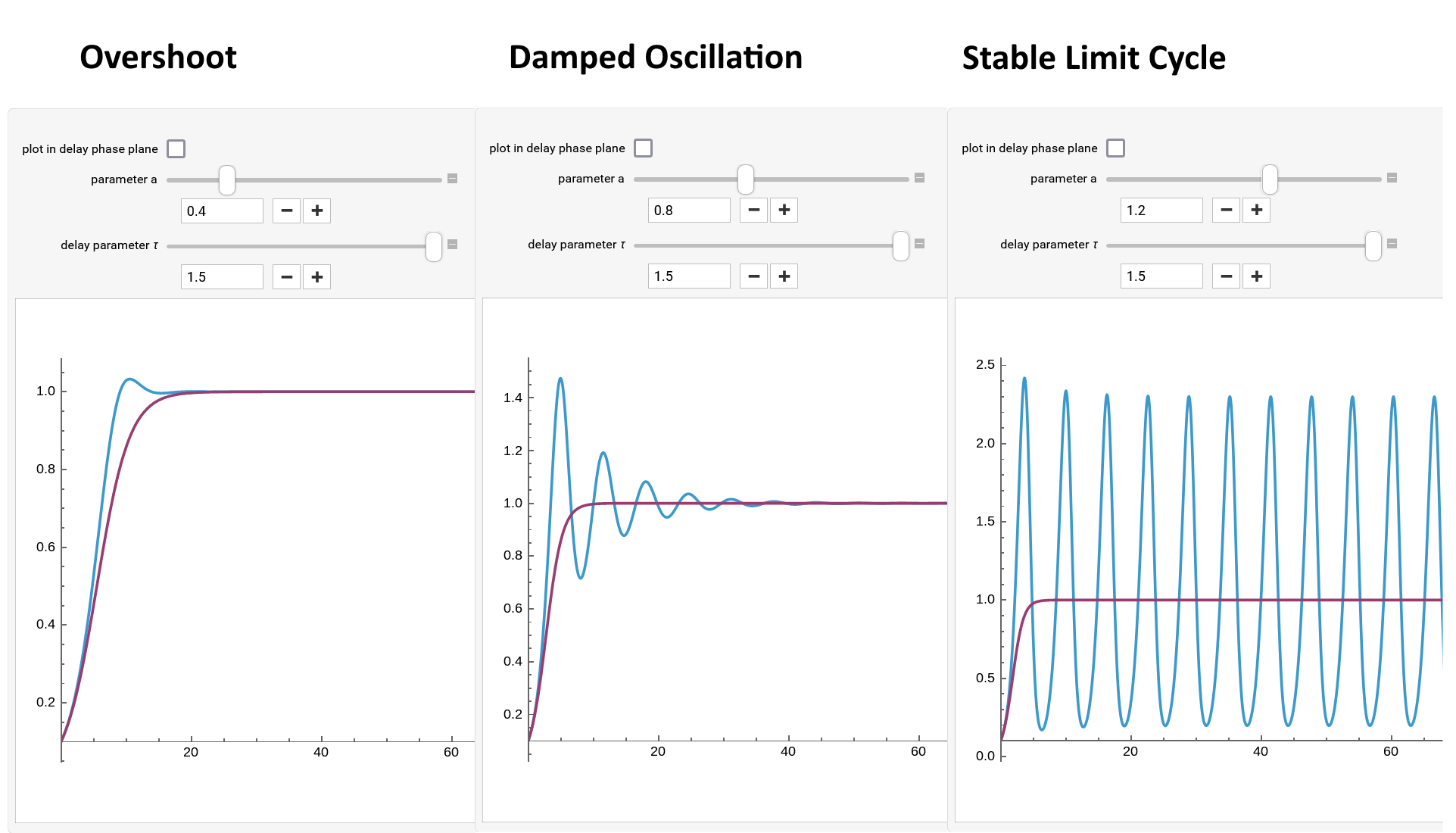 "*stable limit cycle: Like a faulty heating system that always overheats & overcools*" ] --- ## Discrete logistic growth Take discrete population growth equation: `$$N_{t+1} = \lambda N_t$$` break up into "stable" and "growth" components ( `\(\lambda = 1 + r_d\)` ): `$$N_{t+1} = N_t + r_d N_t$$` add the density dependence "brake" on the growth: `$$N_{t+1} = N_t + r_d N_t \left(1 - {N_t \over K}\right)$$` --- ## Discrete Logistic: Two formulations Compute change within a time step: `$$\Delta N_t = r_d N_t \left(1 - {N_t \over K}\right)$$` Compute change of a population: `$$N_{t+1} = N_t + r_d N_t \left(1 - {N_t \over K}\right)$$` These are *difference* (as opposed to *differential*) equations. And ... unlike the differential version ... there is NO "analytical" solution of `\(N_t\)` for any `\(N_t\)`. In fact, this little equation **blew up math as we know it!** > Visit this: https://egurarie.shinyapps.io/DiscreteLogisticMapping/ --- ## Unexpected aspects of chaos theory .... - It emerged from a basic (and old) *deterministic* population model. - It is extremely sensitive to initial conditions - The patterns are fractal <center> <img src='images/logisticmap.png' width='60%'/> </center> --- ## Very nice video on "logistic mapping" .center[ <iframe src="https://www.youtube.com/embed/ovJcsL7vyrk?controls=1" width="70%", height = 400> </iframe> ] --- class: inverse # .Huge[More "Chaotic" dynamics] .center[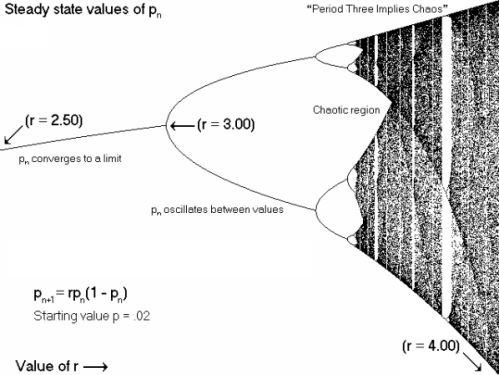] --- .pull-left-60[ ## Single population dynamics: Small Rodents Many, relatively easy long-term studies of small-mammal populations. Observed that populations fluctuate with higher amplitude and different periods / randomness across latitudinal gradients. <img src='images/Lemming.jpg' width='47%'/> <img src='images/KilpisjarviVole.jpg' width='47%'/> Norway lemming *(Lemmus lemmus)* Tundra vole *(Microtus oeconomus)* ] .pull-right-30[ <img src='images/VoleGradient.png' width='100%'/> Turchin and Hanski (1997) ] --- ## Delayed Density Dependence `$$\log(N_t) = a_0 + a_1 \log(N_{t-1}) + a_2 \log(N_{t-2})$$` <center> <img src='images/DelayedDensityDependence.png' width = "60%"> </center> --- ## Lots of data and lots of debate .pull-left-30[ Are we observing deterministic **chaos** or are we observing **stochasticity**? What *IS* chaos in a stochastic world? > But ... methodologically ... it is ALL done by fitting **simple linear models**! ] .pull-right-70.center[ <img src='images/Stenseth_megafigure.png' width = "100%"> Stenseth (1999) *Oikos* ] --- ## Top 10 subsection heading of all time .pull-left-60[ 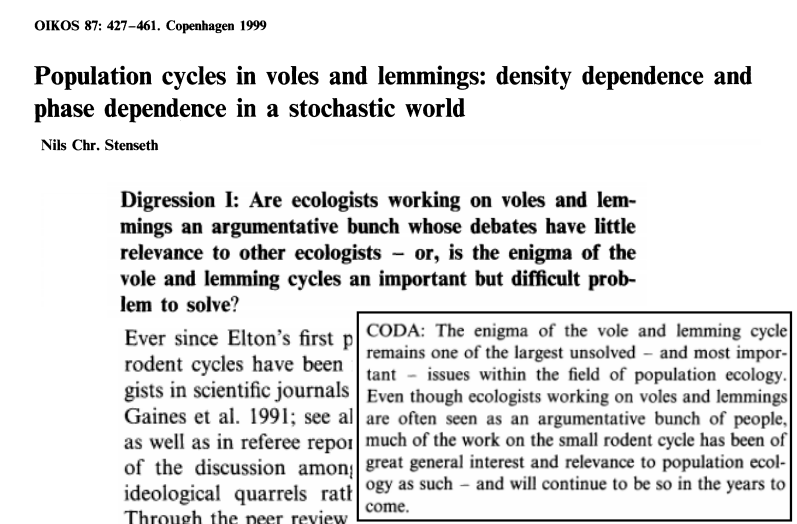 ] -- .pull-right-40.large[ > **My conclusion:** > > Argumentative or not, vole/lemming population ecologists certainly have a high **self-regard**! ] --- ## Next week (and beyond) .... We blow up: `$$\huge N_t$$` .pull-left[ **into:** >- sex / age classes >- multiple sub-populations >- multiple species (competitors / predator-prey) >- infected, susceptible, recovered ] .pull-right[ **relying on:** >- difference and differential equations >- probability and stochasticity >- visualization and simulation >- statistics (esp. linear modeling) >- ***natural history and biological intuition!*** ]