class: left, top, inverse, title-slide .title[ #
Structured populations
] .subtitle[ ##
EFB 370: Population Ecology
] .author[ ### .white[**Dr. Elie Gurarie]** ] .date[ ### .white[March 3, 2025] ] --- ## So far ... `$$\huge N_t = N_{t-1} + B_t - D_t$$` with two assumptions: .pull-left-40.darkblue[ ### Exponential Growth **Birth rates** and **Death rates** constant Total **Births** and **Deaths** proportional to **N** ] .pull-right-60.darkgreen[ ### Density-dependent Growth **Birth rates** decrease and/or **Death rates** increase with **N**. Total **Births** and/or total **Deaths** increase & decrease with **N**. ] -- .center[ **Analysis always relies on:** difference and differential equations | understanding randomness and stochasticity | visualization and simulation | statistics (esp. linear modeling) | ***natural history and biological intuition!*** ] --- ## Coming up in the next weeks we blow up: `$$\huge N_t$$` **into:** | | | |---|----| | sex / age classes: | **structured populations** | | multiple sub-populations: | **meta-populations** | | multiple species: | **competitors / predator-prey** | | infected, susceptible, recovered: | **disease dynamics** | -- **Always relying on:** - difference and differential equations - probability and stochasticity - visualization and simulation - statistics (esp. linear modeling) - ***natural history and biological intuition!*** --- ## Drilling into structure of Birth and Death .darkblue[ `$$\Large N_t = N_{t-1} + B_t - D_t$$` ] .box-blue[ .pull-left[ **B** = Births - **Fecundity** = `\(f\)` <br> `#` births / female / unit time (*unit time* can be any unit of time, but is usually year) ] .pull-right[ **D** = Deaths - **Survival (rate)** = `\(s\)`: probability of surviving / unit time - **Mortality (rate)** = `\(m = 1-s\)` probability of dying in unit time ] ] .pull-left-70[ ### Life History is the **pattern** of **survival** and **reproduction**. **"pattern"** means organized by: Age | Size | Stage (larva / pupa / adult) ] .pull-right-30[ <br> > ### Gotelli - Chapter 3 ] --- # Life history strategies: *r*-selected, vs. *K*-selected species For a long time a popular **paradigm** (conceptual model purporting to explain a wide range of phenomenon) for understanding evolutionary drivers of life-history variation. Still popularly taught: .center[ <iframe src="https://www.youtube.com/embed/Bu6ouKt9zhs?controls=1" width="800" height="450"> </iframe> ] --- background-image: url("images/walrusoysters.png") background-position: center background-size: contain .pull-left-30[ ### r-selected species #### strategy: - lots of offspring - little or no parental investment - semelparous - early maturity - Type III survivorship - low survivorship - short life-expectancy #### drivers: - small size - unstable / unpredictable environments #### consequence - highly fluctuating populations ] .pull-right-30[ ### K-selected species #### strategy: - few offspring - lots of parental investment - high survivorship - late maturity - iteroparous - long life-expectancy - Type I survivorship schedule #### drivers: - stable environments - large #### consequence - more stable / slowly-fluctuating populations ] --- ## Nice theory you've got there, but lots of counter-examples .pull-left[ - What about **trees**? They're big, they're long-lived (very **K**), but they produce and disperse a **heckload** of seeds (very, very **r**). ] .pull-right[ - What about **iteroparous** species (**K**) that are hedging their bets against high inter-annual variation in environmental conditions (very **r**)? ] .pull-left[<br><br>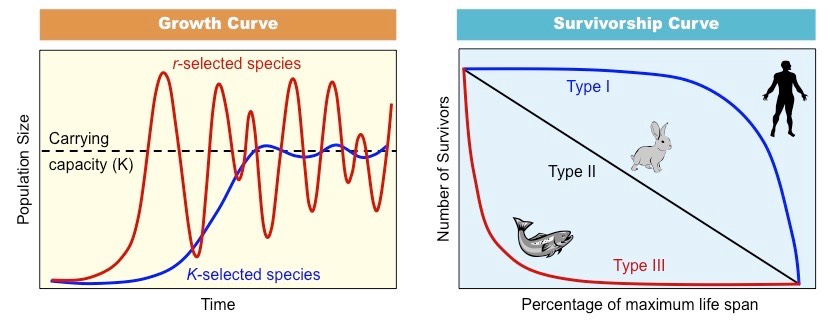] .pull-right[ > .green[The r- and K-selection paradigm was focussed on **density-dependent selection**. This paradigm was challenged as it became clear that ... **age-specific mortality** provide[s] a more mechanistic link between an environment and an **optimal life history** ...] ([Reznick et al. (2002) Ecology](https://esajournals.onlinelibrary.wiley.com/doi/full/10.1890/0012-9658%282002%29083%5B1509%3ARAKSRT%5D2.0.CO%3B2)) ] --- background-image: url("images/salmonids.png") background-size: cover ## Salmonid (counter)-example <br><br><br> .center.large[ **Semelparous species** Much bigger eggs (189 > 86 mg). Also nest building and guarding behavior, before dying, i.e. greater investment in **Juvenile Survival** over **Adult Survival**. The iteros just keep staying alive and trying to breed again and again. ] -- .pull-left.center[ > Inconsistent with **r**-**K** paradigm! ] --- .pull-left[ ### Tasmanian devil (*Sarcophilus harrisii*) .center[<img src='images/tazzy.jpg' width='70%'/>] Only marsupial carnivore | range restricted to Tasmania .pull-left[ .darkred[Dying of *facial tumor disease*; an infectious cancer (!) which kills nearly all adults > 3 years ]] .pull-right[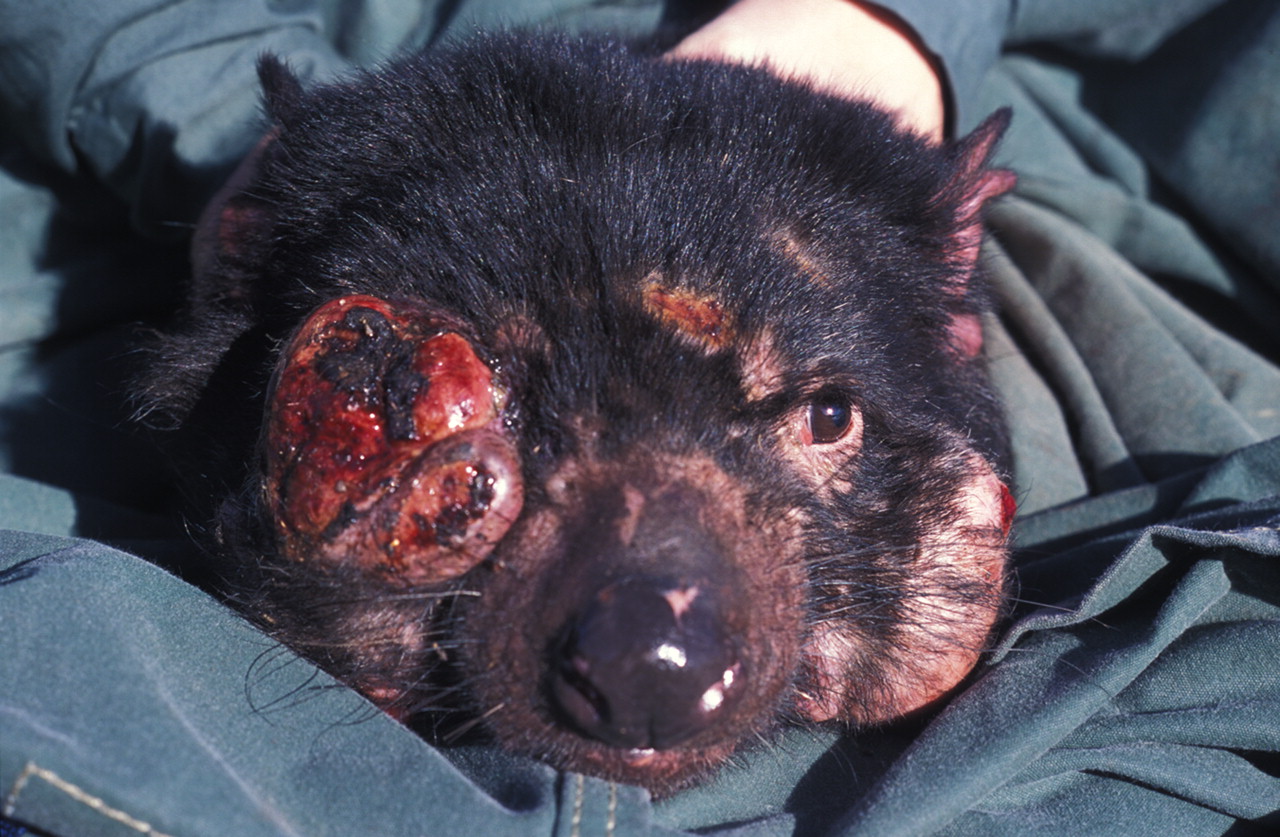] ] -- .pull-right-40[ ### Switch to Semelparity .green[**Previously:** Longer-lived, and iteroparous, with later birth (over 1 year old)] 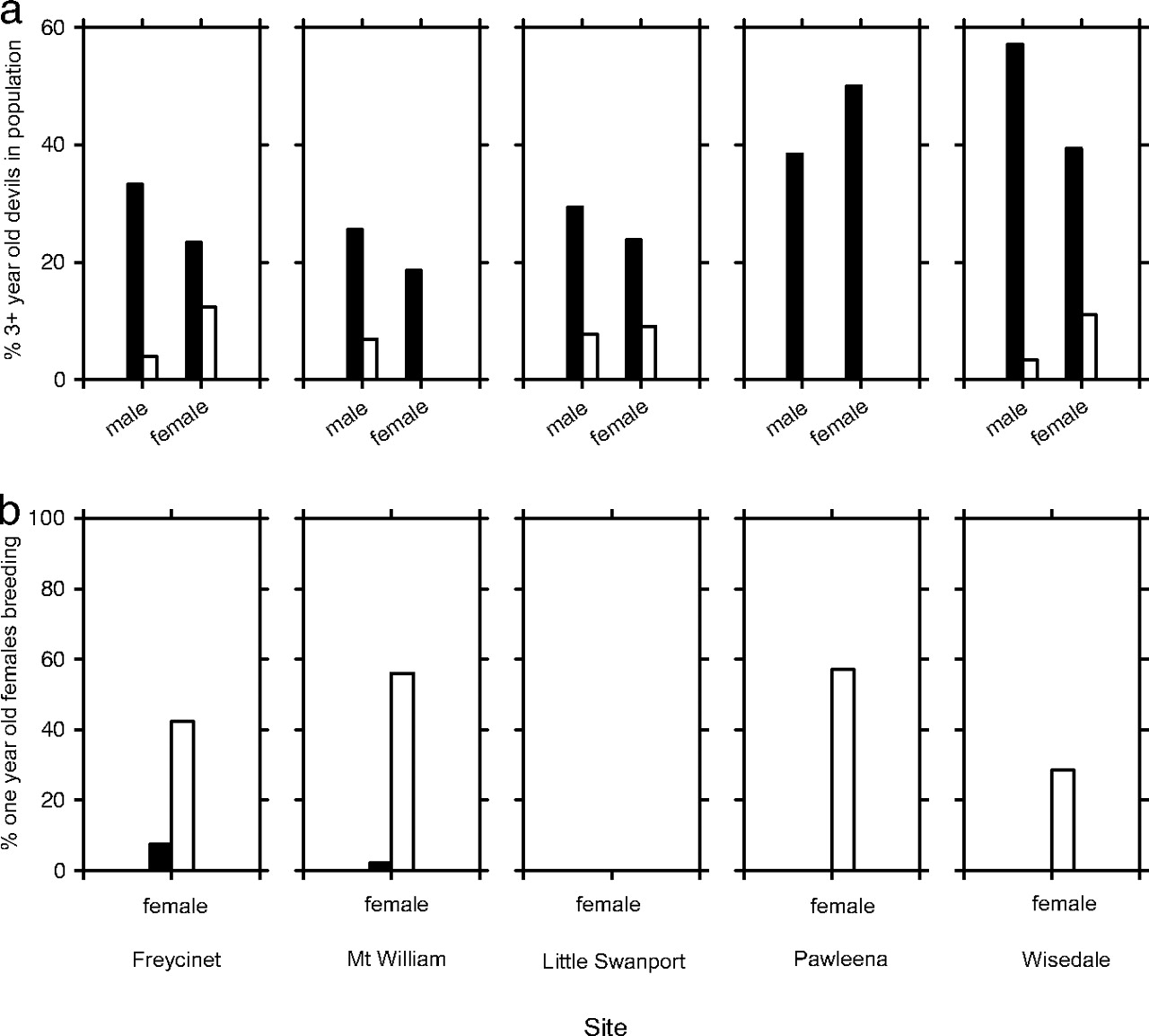 .darkblue[**Now:** Semelparous, one-shot, younger mothers (almost NO 2-3 year old animals!)] ([Jones et al. (2013)](https://doi.org/10.1073/pnas.0711236105)) ] --- .pull-left-60[ ## **Basic fact of life I:** Survival varies with age! 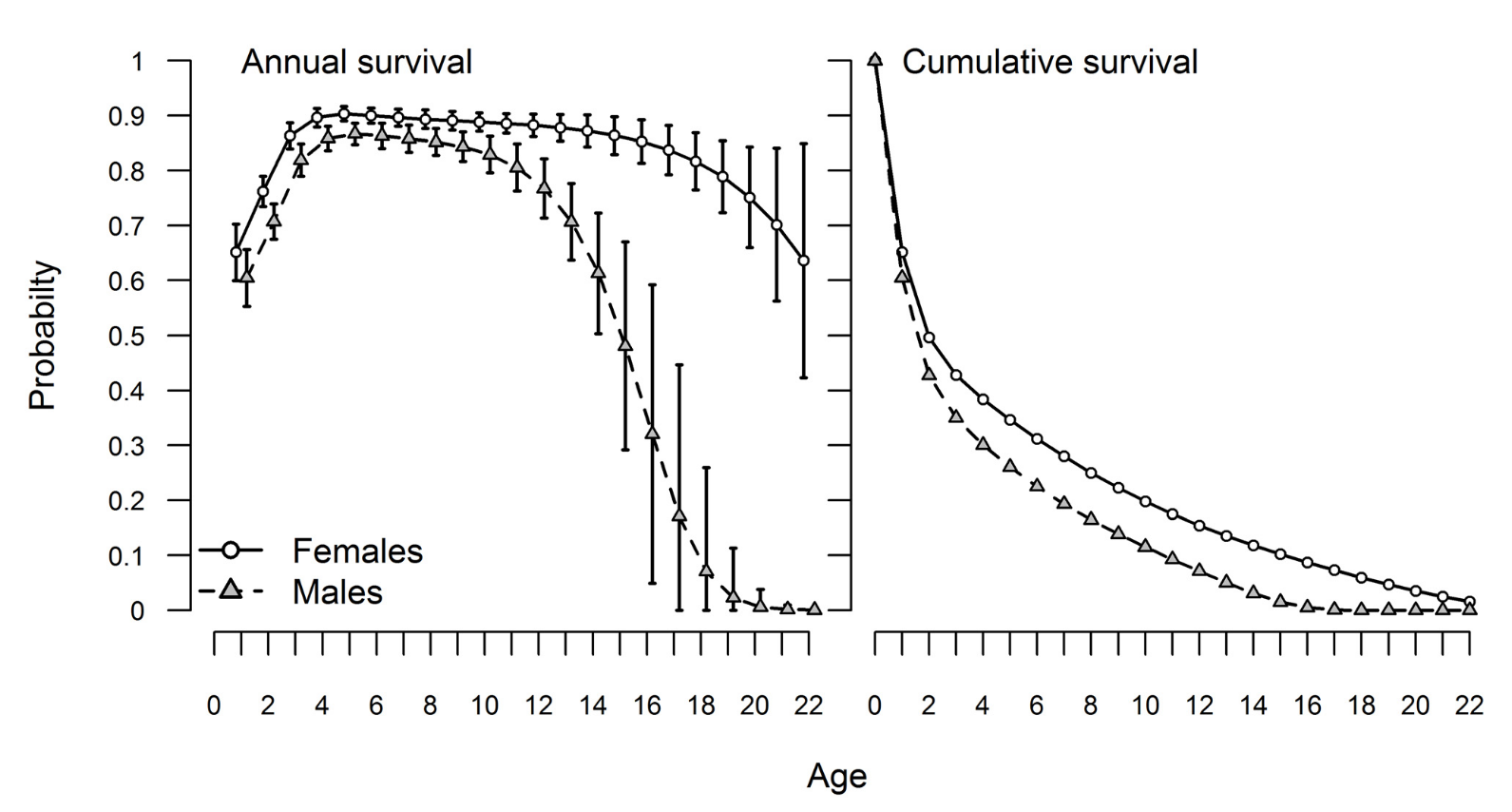 - **Survival Probability** ( `\(S_0, S_1, S_2, ...\)` ) always between 0 and 1. - **Cumulative Survival** ( `\(1, S_0, S_0 S_1, S_0 S_1 S_2, ...\)` ) always starts at 1 and goes to 0 .center[([Altukhov et al. 2015](https://journals.plos.org/plosone/article?id=10.1371/journal.pone.0127292) )] ] .pull-right-40[ 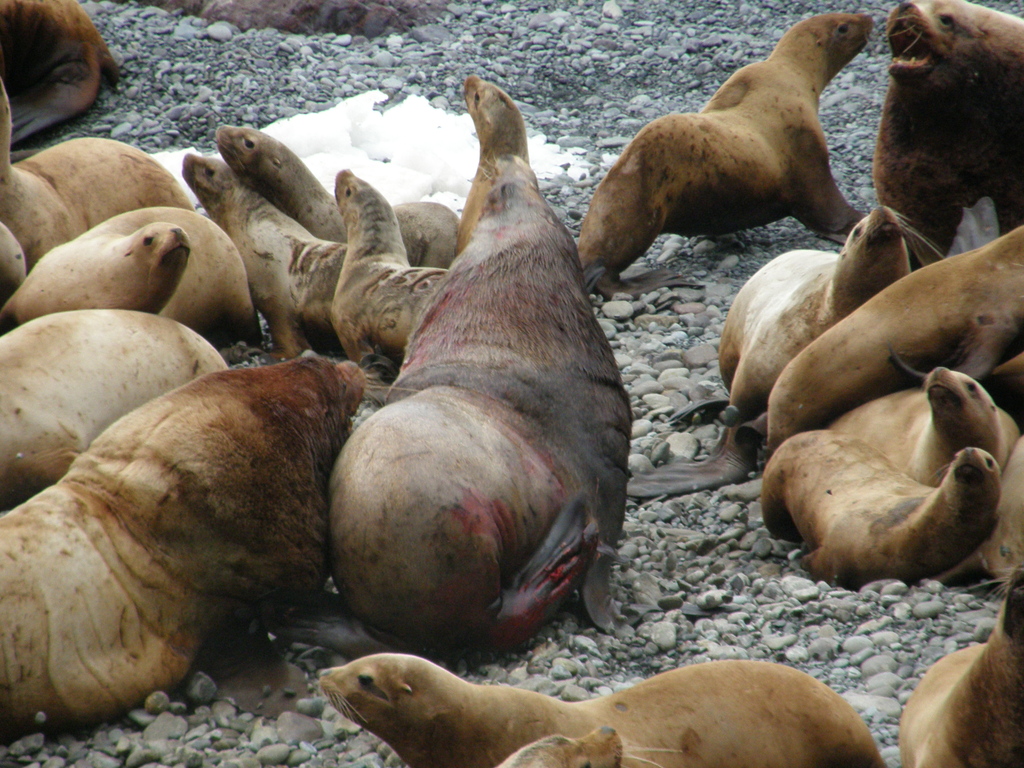 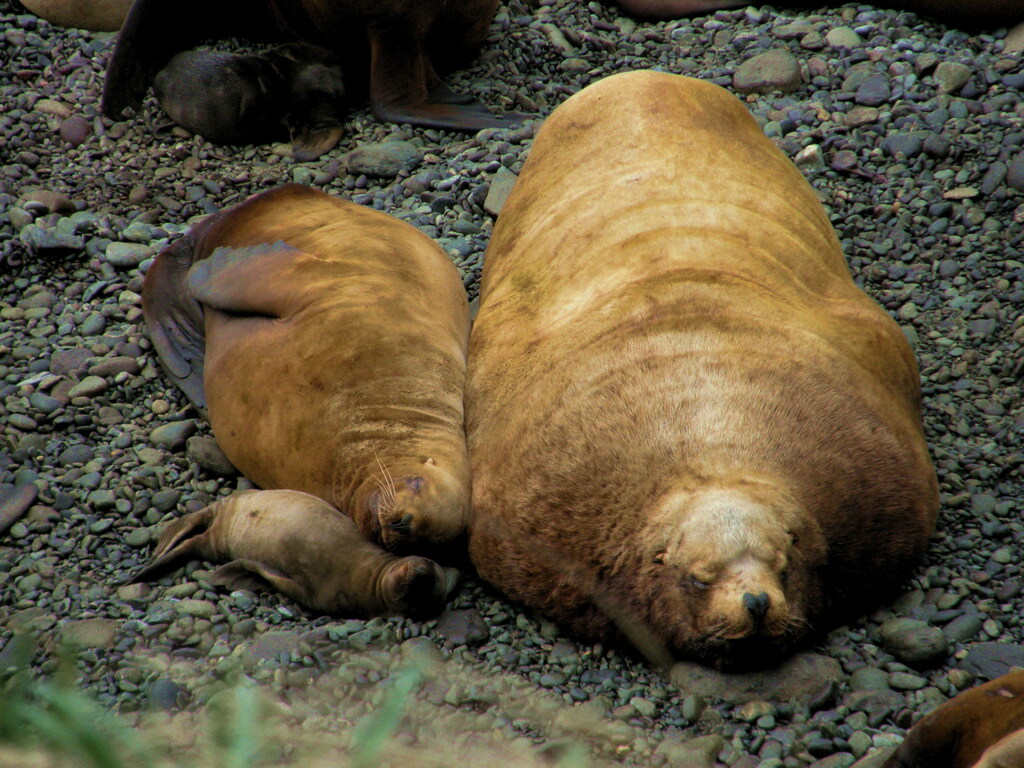  ] --- ## **Basic fact of life II:** Fecundity varies with age! 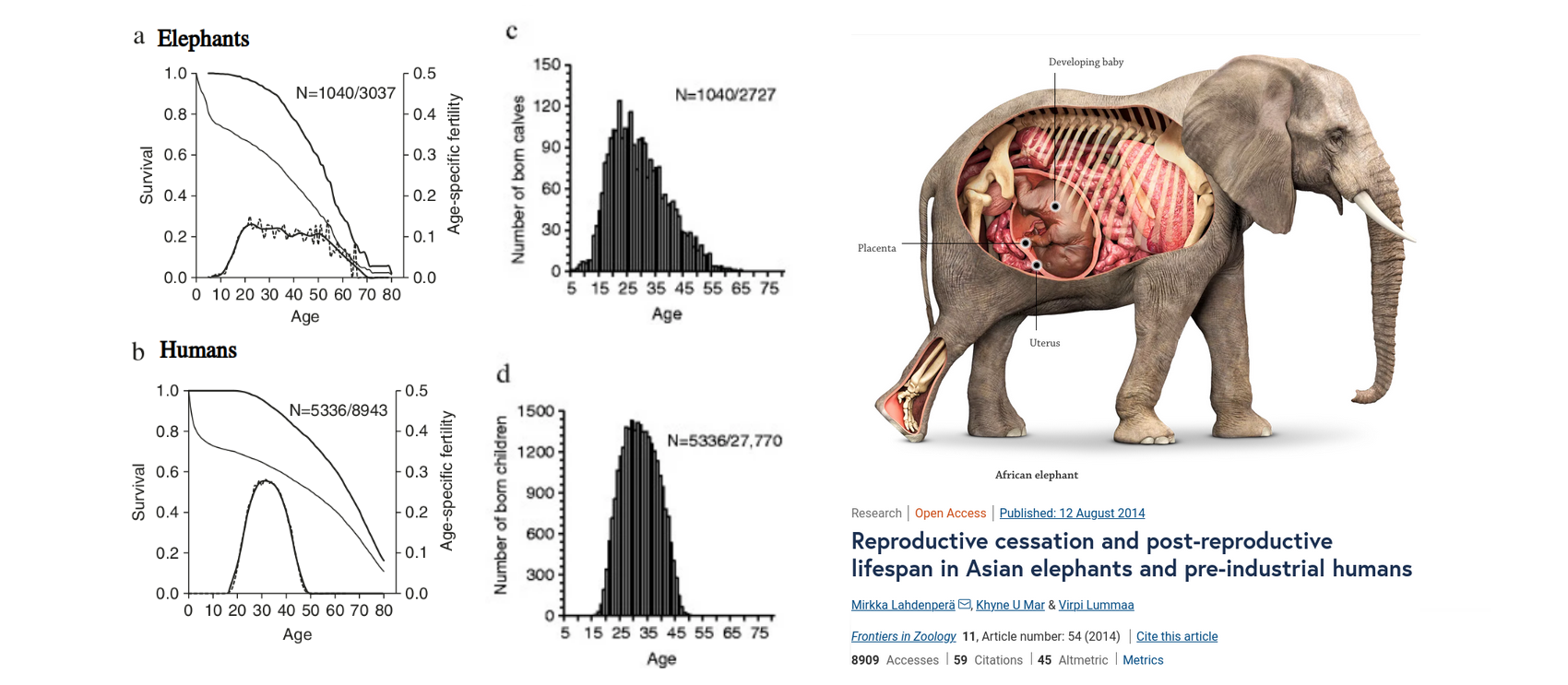 .center[([Lahdenperä et al. 2015](https://frontiersinzoology.biomedcentral.com/articles/10.1186/s12983-014-0054-0))] --- ## *Monoceros academicus*: Three Life Stages . | Larva | Sophomore | Emeritus | --- |:----------:|:------:|:---:| . | <img src='images/unicornlarva.webp' height='150px'/> | <img src='images/unicornstudent.jpg' height='150px'/> | <img src='images/oldunicorn.webp' height='150px%'/> | <font size = 5> Survival | <font size = 5> 0.5 |<font size = 5>1 | <font size = 5>0 | <font size = 5> Fecundity |<font size = 5> 0 | <font size = 5>1.5 | <font size = 5>0.5 | |||| > - **Survival** is a **probability** (unitless) > > - **Fecundity** is an **expected number** of offspring (n. ind.). </center> -- **Human experiment:** 16 volunteers please. --- .pull-left-40[ ## Experiment: results Stage | Survival | Fecundity ----------|------|--- 1. larvae | 0.5 | 0 2. sophomore | 1 | 1 3. emeritus | 0 | .5 See numerical experiment: https://egurarie.shinyapps.io/AgeStructuredGrowth/ ] .pull-right-60[ <img src="PopulationStructure_PartI_files/figure-html/runPop1-1.png" width="100%" /> >- Eventually obtains a 2:1:1 stable age distribution >- Overall growth = 1 ] --- .pull-left-40[ ## Change one value .... Stage | Survival | Fecundity ----------|------|--- 1. larvae | 0.5 | 0 2. sophomore | 1 | <font size = 6, color = "red"> **2** </font> 3. emeritus | 0 | .5 ] .pull-right-60[ <img src="PopulationStructure_PartI_files/figure-html/runPop2-1.png" width="100%" /> > This time, population grows. > And the stable age distribution is a bit different. ] --- class: inverse .pull-left-40.large[ ## We want to know: - What is the **population growth rate**? - What is the **stable age distribution**? - How sensitive are these to variation in **age-structured** survival and fecundity? ] -- .pull-right-60.center[ Our secret weapon in this quest .... ## .Large[**Matrices!**] 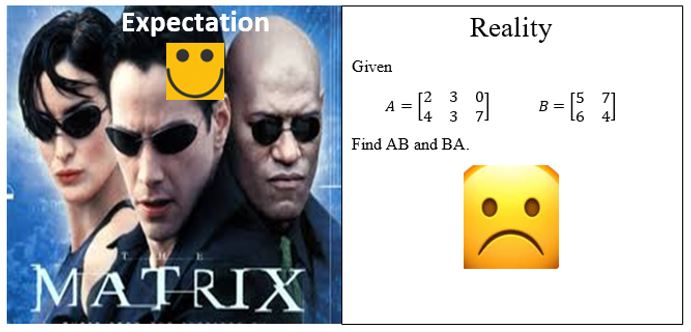 ] --- # Matrix population modelling ## Total populations vs. structured populations .pull-left[ .large[**Scalar:**] `$$\huge N_t$$` ] .pull-right[ .large[**Vector:**] `$$\large \vec{N}_t = \begin{cases}n_{1,t} \\ n_{2,t} \\ n_{3,t} \\ \vdots \\ n_{k,t}\end{cases}$$` ] --- ## Exponential Growth - Structured Population Growth .pull-left[ **Exponential Growth** `$$\huge N_{t+1} = \lambda N_t$$` `\(\lambda\)` is a scalar population growth multiplier ] .pull-right[ **Structured Population Growth** `$$\huge \vec{N}_{t+1} = \textbf M \vec{N_t}$$` `\(M\)` is a **Matrix** population **structure** growth multiplier ] --- ## Population transition (aka **Leslie**) matrix A **matrix** is a tool for **transforming vectors**. A **population matrix** transforms a structured population **vector** by 1. adding newborns, [fecundity: `\(f_i\)`] 2. killing off older classes, [survival: `\(s_i\)`] 3. scootching everyone up the stage ladder [aging] .pull-left[ `$$\begin{bmatrix} n_0 \\ n_1 \\ \vdots \\ n_{\omega - 1} \\ \end{bmatrix}_{t+1} = \begin{bmatrix} f_0 & f_1 & f_2 & \ldots & f_{\omega - 2} & f_{\omega - 1} \\ s_0 & 0 & 0 & \ldots & 0 & 0\\ 0 & s_1 & 0 & \ldots & 0 & 0\\ 0 & 0 & s_2 & \ldots & 0 & 0\\ \vdots & \vdots & \vdots & \ddots & \vdots & \vdots\\ 0 & 0 & 0 & \ldots & s_{\omega - 2} & 0 \end{bmatrix} \begin{bmatrix} n_0 \\ n_1 \\ \vdots\\ n_{\omega - 1} \end{bmatrix}_{t}$$` ] .pull-right-40[ **The Leslie matrix** - is square - the rows and columns represent age classes - the top row is the number of **births** coming in from older age classes - the lower rows are the number of **survivors** into the next age class ] --- ## Remember *Monocerus academicus* ... .pull-left-60.center[ . | Larva | Sophomore | Emeritus | --- |:----------:|:------:|:---:| **Monoceros academicus** | <img src='images/unicornlarva.webp' height='100px'/> | <img src='images/unicornstudent.jpg' height='100px'/> | <img src='images/oldunicorn.webp' height='100px%'/> | Survival | 0.5 |1 | 0 | Fecundity | 0 | 1.5 | 0.5 | ] .pull-right-40[ Here's the matrix: $$ \large M = \\begin{bmatrix}0&1.5&0.5 \\\\0.5&0&0 \\\\0&1&0 \\\\\\end{bmatrix} $$ ] --- ## Leslie matrix as diagram The population matrix maps to a diagram! These are very useful: .pull-left-30[ ### Matrix $$ \Large M = \\begin{bmatrix}0&1.5&0.5 \\\\0.5&0&0 \\\\0&1&0 \\\\\\end{bmatrix} $$ ] .pull-right-70[ ### Diagram 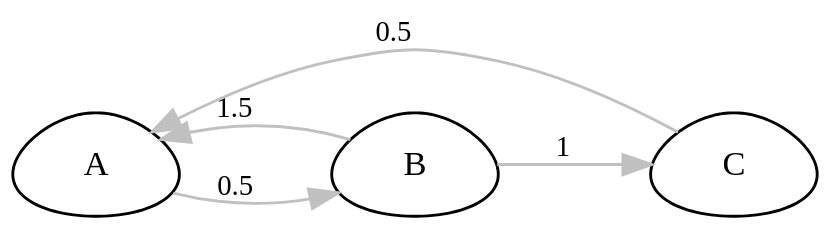 ] .box-grey[ - Arrow **A** to **B** (0.5) is represented by matrix entry **column 1** to **row 2** - survival to second stage. - Arrow **B** to **C** (1) is matrix entry **column 2** to **row 3** - survival to last stage. ] --- ## Ok, but how does it work? Matrix Multiplication looks scary! `$$\begin{align*} M V^{T}= \left[ \begin{array}{cccc} a_{11} & a_{12} & \ldots & a_{1n}\\ a_{21} & a_{22} & \ldots & a_{2n}\\ \vdots & \vdots & \ddots & \vdots\\ a_{n1} & a_{n2} & \ldots & a_{nn} \end{array} \right] \left[ \begin{array}{c} x_1\\ x_2\\ \vdots\\ x_n \end{array} \right] = \left[ \begin{array}{c} a_{11}x_1+a_{12}x_2 + \cdots + a_{1n} x_n\\ a_{21}x_1+a_{22}x_2 + \cdots + a_{2n} x_n\\ \vdots\\ a_{n1}x_1+a_{n2}x_2 + \cdots + a_{nn} x_n\\ \end{array} \right]. \end{align*}$$` But it basically just takes each element of your vector (e.g. population at a stage) and tracks how it is affected by all the other stages. --- ## Start with our experiment: 8 larvae `\(N_0 = \left[\begin{array}{c} 8 \\ 0 \\ 0 \\ \end{array}\right]\)` `$$N_1 = M N_0^T = \begin{bmatrix}0&1.5&0.5 \\ 0.5&0&0 \\0&1&0 \end{bmatrix} \times \left[\begin{array}{c} 8 \\ 0 \\ 0 \\ \end{array}\right] = \left[\begin{array}{c} 0 + 0 + 0 \\ 4 + 0 + 0 \\ 0 + 0 + 0 \\ \end{array}\right] = \left[\begin{array}{c} 0\\4\\0 \\ \end{array}\right]$$` `$$N_2 = M N_1^T = \begin{bmatrix}0&1.5&0.5 \\ 0.5&0&0 \\0&1&0 \end{bmatrix} \times \left[\begin{array}{c} 0 \\ 4 \\ 0 \\ \end{array}\right] = \left[\begin{array}{c} 6 \\ 0 \\ 4 \end{array}\right]$$` `$$N_3 = M N_2^T = M \times \left[\begin{array}{c} 6 \\ 0 \\ 4 \\ \end{array}\right] = \left[\begin{array}{c} 2 \\ 3 \\ 0 \end{array}\right]$$` etc. --- .pull-left-30[ ## Super simple in R! ] .pull-right-70[ ```r M <- rbind(c(0,1.5,.5), c(.5,0,0), c(0,1,0)) row.names(M) <- c("larvae", "sophs", "emeriti") ``` ] .pull-right-30[ ] .pull-left-40[ One step: ```r M %*% N0 ``` ``` ## [,1] ## larvae 0 ## sophs 9 ## emeriti 0 ``` Two steps: ```r M %*% M %*% N0 ``` ``` ## [,1] ## larvae 13.5 ## sophs 0.0 ## emeriti 9.0 ``` ] .pull-right-40[ Three steps: ```r M %^% 3 %*% N0 ``` ``` ## [,1] ## larvae 4.50 ## sophs 6.75 ## emeriti 0.00 ``` 20 steps: ```r M %^% 20 %*% N0 ``` ``` ## [,1] ## larvae 8.000124 ## sophs 3.999882 ## emeriti 4.000225 ``` ] --- ## Plotting the results `$$N_t = M^t \times N_0$$`
Structured population ...
(if no animation shows up, click [here](StructuredPop.html)) --- ## Amazing property of (many) matrices ... <br><br> `$$\huge M \times N^* = \lambda N^*$$` .large[ For every (.darkgreen[**population**]) **matrix** there is a **vector** (.green[**age distribution**]) for which the matrix transformation (.green[**population growth process**]) increases the **vector** by a fixed proportion `\(\lambda\)` (.green[**population growth rate**]). - `\(\large N^*\)` - is the **eigenvector** = .red[**stable population distribution**] - `\(\large \lambda\)` - is the **eigenvalue** = .red[**population growth factor**] ] --- ## Some simple examples (to work out by hand) .pull-left[ <img src='diag0.png' width='100%'/> $$ \large M = \\begin{bmatrix}2&0 \\\\0&2 \\\\\\end{bmatrix} $$ .large[ Everything doubles! - `\(\lambda = 2\)` - no unique distribution. ]] .pull-right[ <img src='diag1.png' width='100%'/> $$ \large M = \\begin{bmatrix}0&2 \\\\1&1 \\\\\\end{bmatrix} $$ .large[ *Eventually* everything doubles! - `\(\lambda = 2\)`. - stable distribution = `\(N^* = \{1, 1\}\)` ]] --- ## *Monocerus academicus* .center[<img src='images/monodiagram.png' width = '50%' />] .pull-left[ ```r matA <- rbind(c(0, 1.5, .5), c(.5, 0, 0), c(0, 1, 0)) ``` $$ \large M = \\begin{bmatrix}0&1.5&0.5 \\\\0.5&0&0 \\\\0&1&0 \\\\\\end{bmatrix} $$ ] .pull-right[ ```r eigen(matA) ``` ``` ## eigen() decomposition ## $values ## [1] 1.0 -0.5 -0.5 ## ## $vectors ## [,1] [,2] [,3] ## [1,] -0.8164966 -0.4082483 0.4082483 ## [2,] -0.4082483 0.4082483 -0.4082483 ## [3,] -0.4082483 -0.8164966 0.8164966 ``` - First eigenvalue: `\(\lambda = 1\)` - Ratio of first eigenvector: 50% : 25% : 25% ] --- ## What if some emeriti decide to go back to school? <center> <img src='diag_backtoschool.png' width = '50%' /> </center> .pull-left-60[ $$ \large M = \\begin{bmatrix}0&1.5& 0.5 \\\\0.5&0&\color{red}{\textbf 0.5} \\\\0&1&0 \\\\\\end{bmatrix} $$ ] .pull-right-40[ ```r eigen(matB) ``` ``` ## eigen() decomposition ## $values ## [1] 1.2071068 -1.0000000 -0.2071068 ## ## $vectors ## [,1] [,2] [,3] ## [1,] 0.7736915 0.5773503 0.6669930 ## [2,] 0.4878914 -0.5773503 0.1511012 ## [3,] 0.4041824 0.5773503 -0.7295812 ``` ] <br> <br> .large[ - First eigenvalue: `\(\lambda = 1.21\)` - Ratio of first eigenvector: 46% : 29% : 24% ] --- ## How does this look? .center[
Back To School ...
(If animation does not work, click [here](BackToSchool.html)) ] --- ### Complex life histories .pull-left-60[ 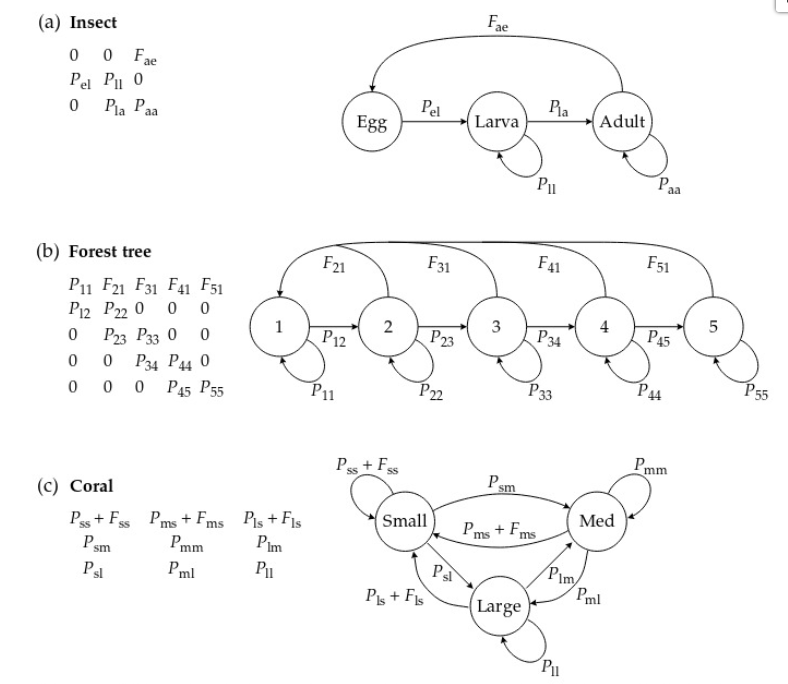 ] .pull-right-30[ .large[ .blue[ #### In short ... ... matrix models do it all! And do it fast, and intuitively. ] ]]