class: center, top, title-slide .title[ #
Population Ecology Part I
] .subtitle[ ## .white[EFB 390: Wildlife Ecology and Management] ] .author[ ###
Dr. Elie Gurarie
] .date[ ### October 19, 2023 ] --- <!-- https://bookdown.org/yihui/rmarkdown/xaringan-format.html --> ## Meet ... *Enhydra lutris* .pull-left[ 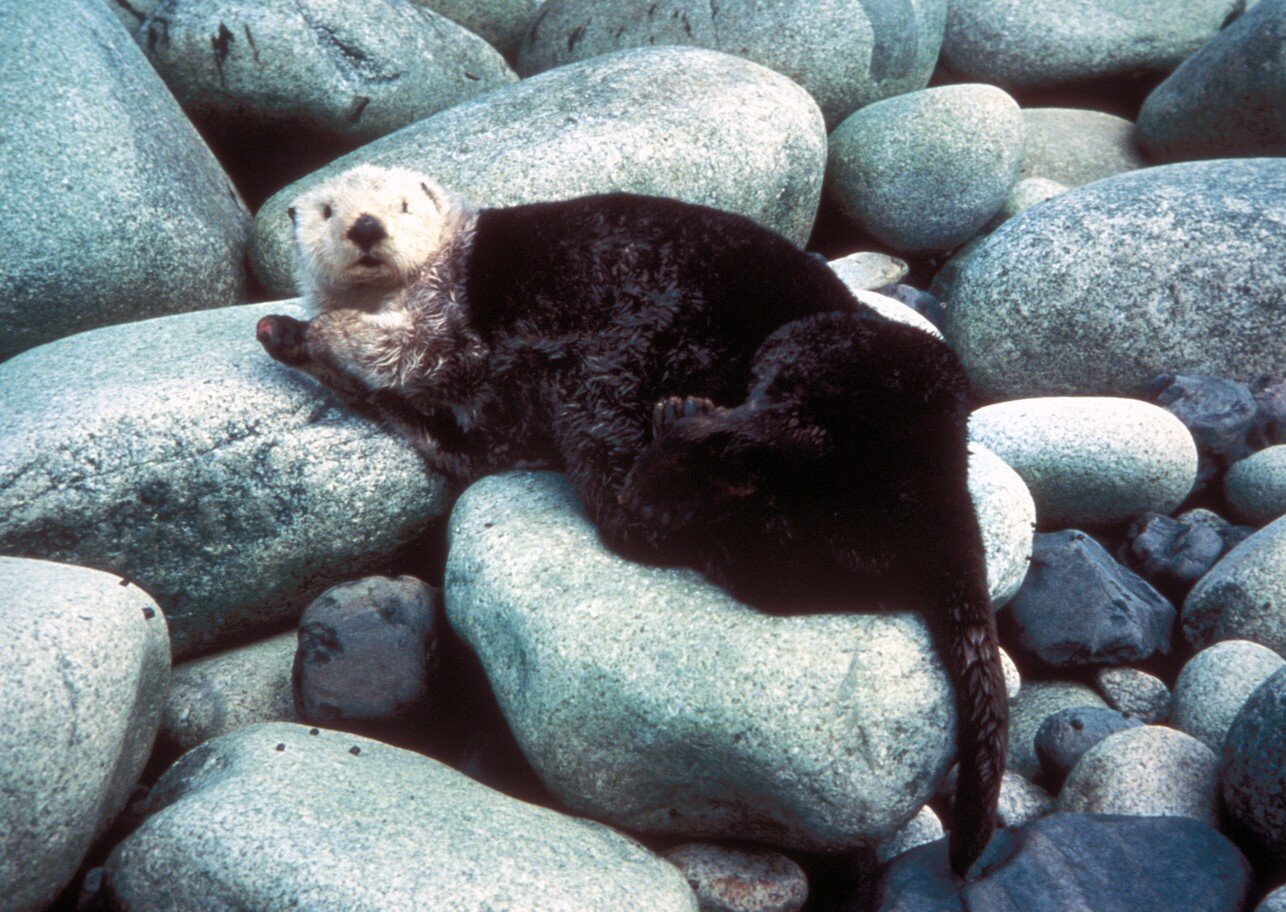 ] .pull-right[ - The largest ... - The smallest ... - The furriest ... * guess how may hairs per in^2^? (*hint, humans are born with ~100,000 TOTAL.*) ] --- ## Sea otters: Range .pull-left-60[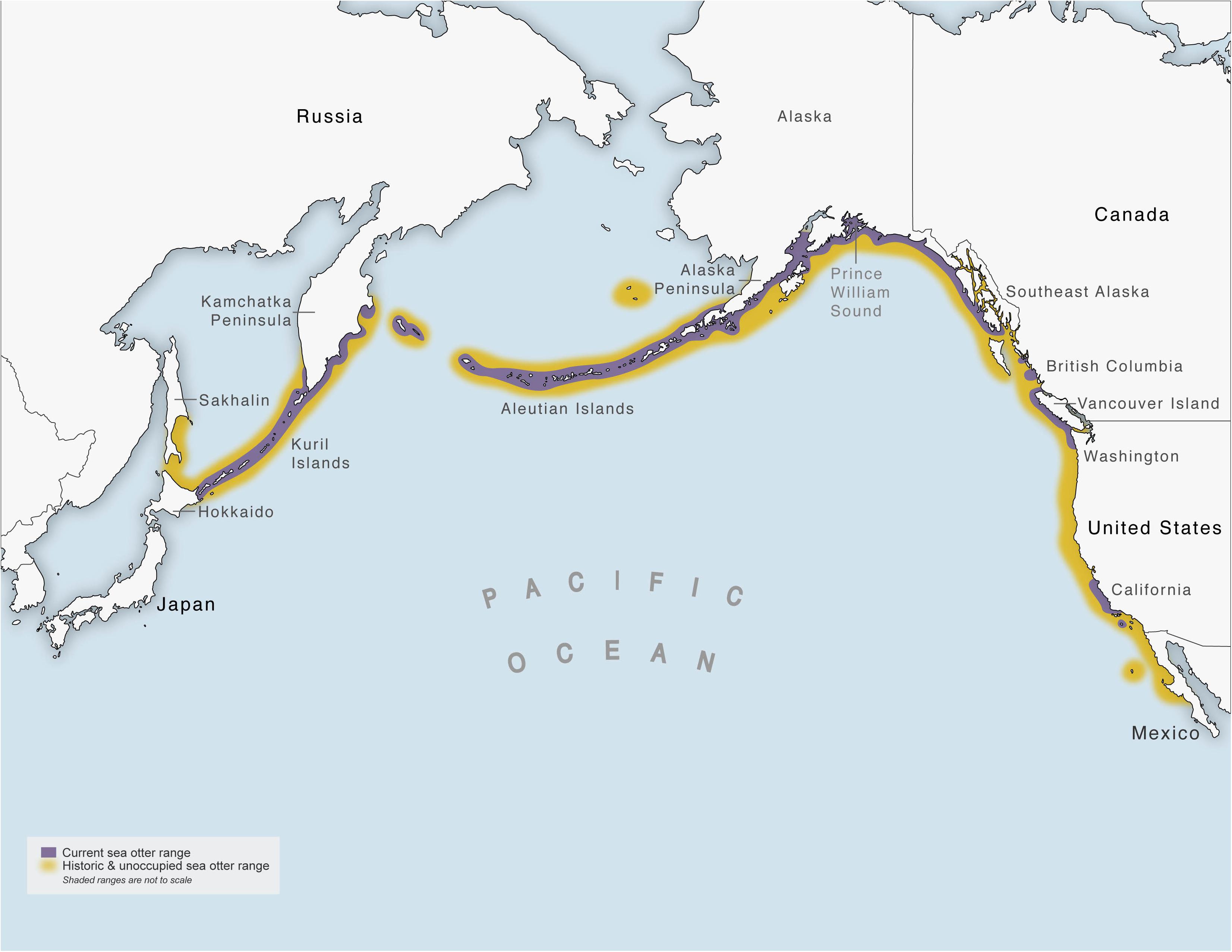] .pull-right-40[*Littorally* the entire North Pacific] --- ## Sea otters: Keystone Species 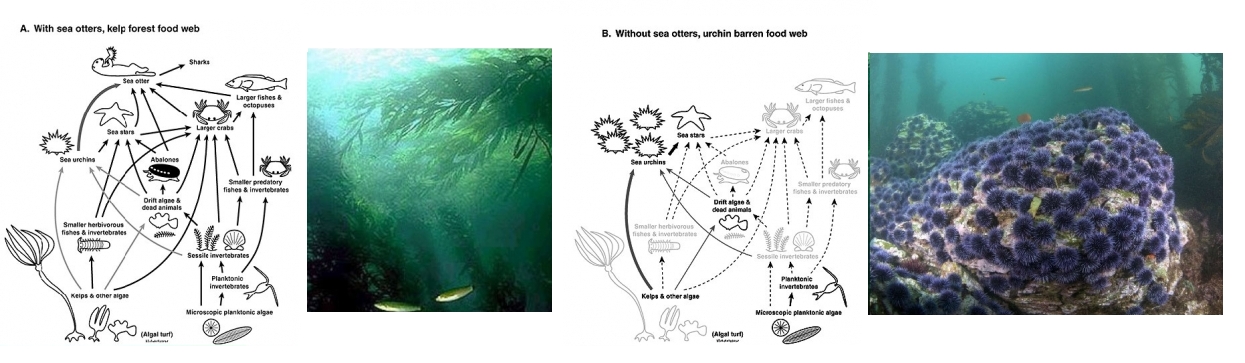 (Estes et al. 1974) --- .pull-left-60[ ## Sea otters: Furriness > Cuteness 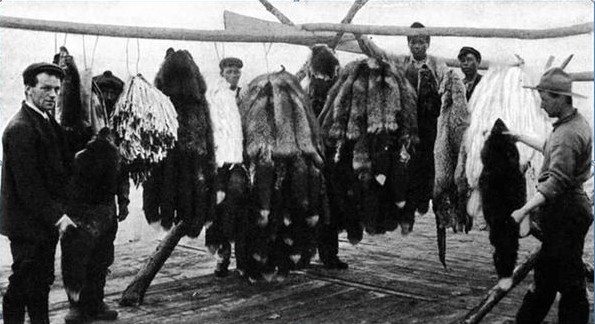 ] .pull-right-30[ 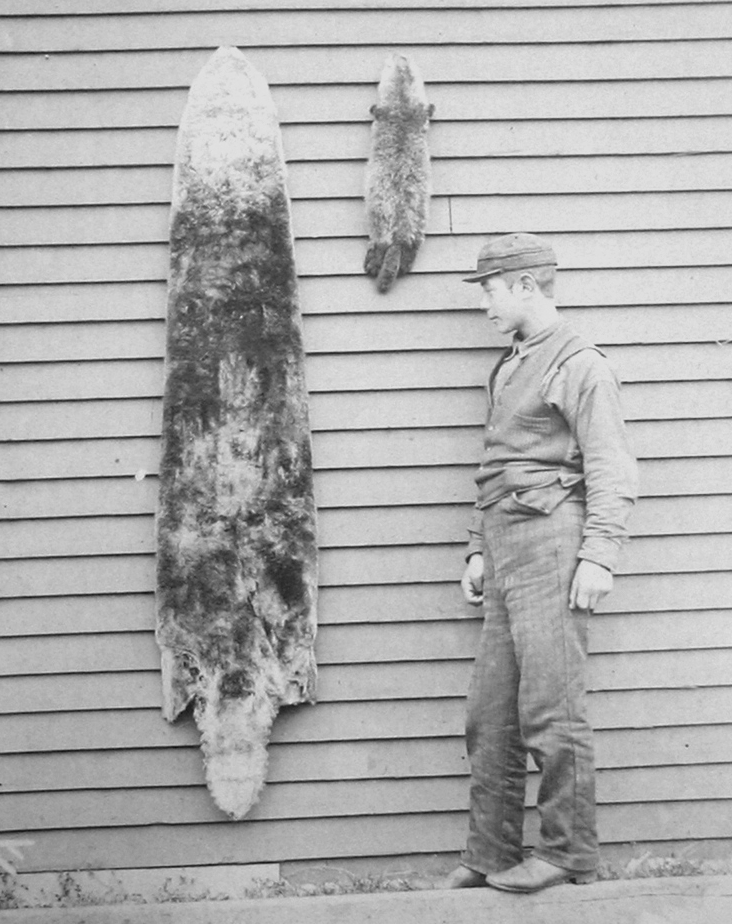 ] .pull-left[ - Fur trade (Russian -> British -> American) leads to near **extirpation** across the entire range. - **> 300,000 in 1740 ... < 2,000 in 1900**. - Displacement and indenturing of Indigeneous fishermen (esp. Aleut) ] .pull-right[ > .footnotesize[*... the rush for the otters’ “soft gold” was a predictable boom and bust ... a cautionary example of unsustainable resource use, and a socioeconomic driver of Western — mainly American — involvement in the Pacific region,* (Loshbaugh 2021)] ] --- ## Sea otter reintroduction: Pacific NW Remnant populations from Aleutian Islands ... released in OR, WA, BC and SE-AK 1969 – 1972. .pull-left[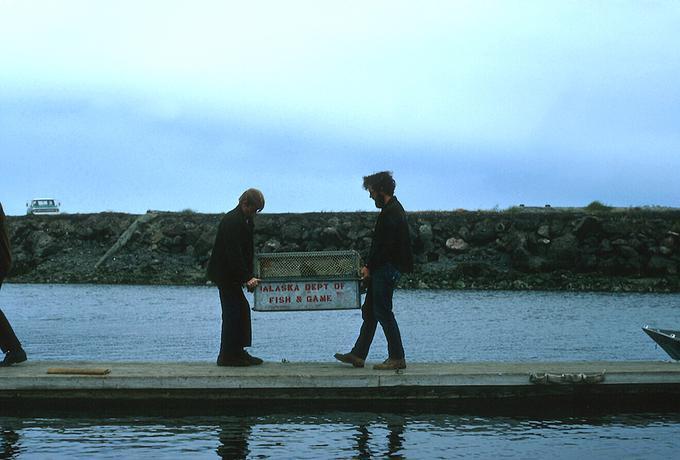] .pull-right[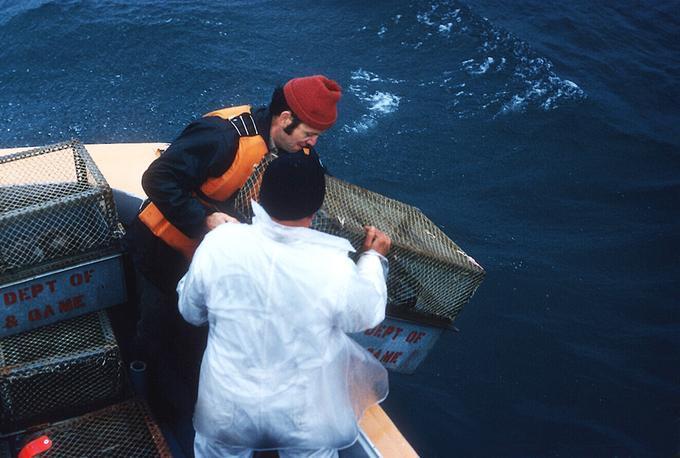] --- ## Sea otter reintroduction: Washington State ... .pull-left[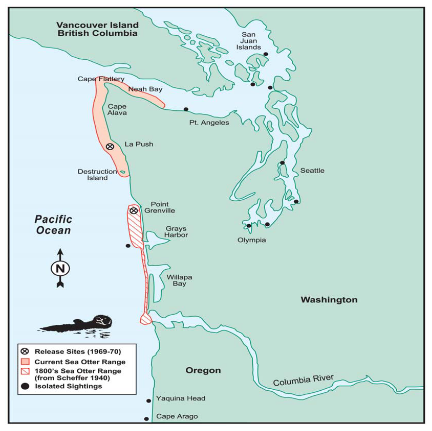] .pull-right[ 1970: 60 otters 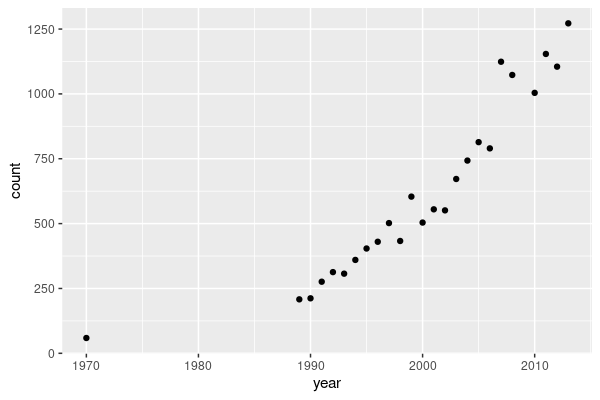<!-- --> 2010's: over 1000 ] .center[***Successful!***] --- ## Population ecology is all about ... `$$\huge N$$` but where? when? -- ## Here! Now! .. `$$\huge N_t$$` but how many were there? --- ## That many, then `\(\Delta t\)` ago! `$$\Large N_t = N_{t - \Delta t} + \Delta N$$` slight rearrangement: `$$\Large N_{t+1} = N_t + \Delta N$$` For now, `\(\Delta t = 1\)`, i.e. it's the discrete unit that we measure population change. VERY TYPICALLY - whether because of biology or field seasons: `$$\Delta t = 1\,\, \textrm{year}$$`. --- ## How does population change? `$$\Large N_{t+1} = N_t + (B - D) + (I - E)$$` ### **B**irth ### **D**eath ### **I**mmigration ### **E**migration --- ## Assumption 1: no one's getting on or off the bus `$$\Large N_{t+1} = N_t + B - D$$` ### **B**irth ### **D**eath ### .red[**I**mmigration] ### .red[**E**migration] This is a **closed population** ... --- ## Assumption 2: the important one The number of *Births* and *Deaths* is proportional to *N*. `$$\Large N_{t+1} = N_t + bN_t - dN_t$$` What does that mean? .pull-left[ - Every female gives birth to the same number of offspring? - Every female has the same *probability* of giving birth? - Every female has the same *probability* of giving birth to the same *distribution* of offspring? ] .pull-right[ - A fixed proportion of all individuals dies? - Every individual has the same *probability* of dying? - the *distribution* of probabilities of dying is constant? ] --- ## Some math .... Define `$$r_0 = b - d$$` `\(r_0\)`: **discrete growth factor**, `$$N_{t+1} = N_t + r_0 N_t$$` `$$N_{t+1} = (1 + r_0) N_t$$` `$$N_{t+1} = \lambda N_t$$` `\(\lambda\)` is .blue[**rate of growth (or decrease)**] - If `\(d > b\)`, `\(\lambda < 1\)`. - If `\(b > d\)`, `\(\lambda > 1\)`. --- ## Cranking this forward `$$N_{t+1} = \lambda(N_t)$$` `$$N_{t+2} = \lambda(N_{t+1}) = \lambda^2 N_t$$` `$$N_{t+3} = \lambda^3 N_t$$` Solution: `$$\large N_{t+y} = \lambda^y N_t$$` or `$$\huge N_t = \lambda^t N_0$$` .center[***Geometric*** (same as ***Exponential***) growth.] --- ## Some examples 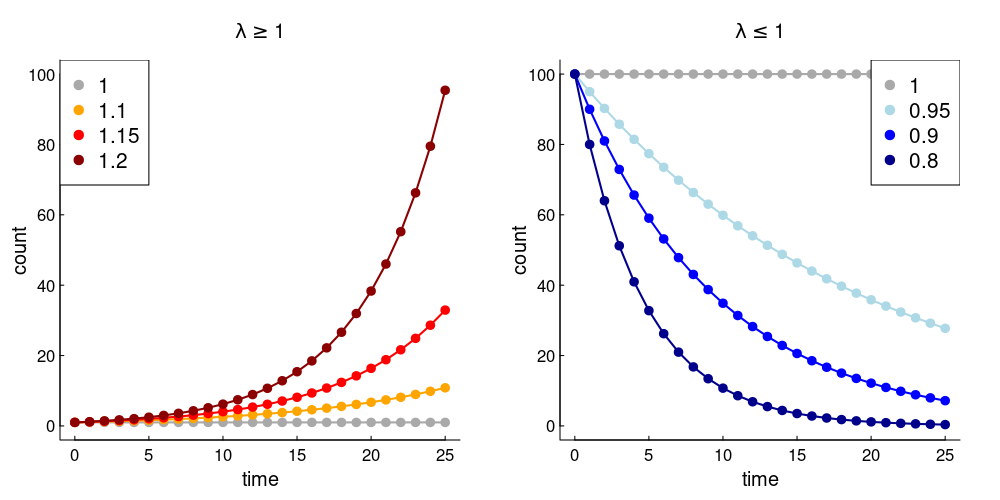<!-- --> --- ## How fast is exponential/geometric growth? .pull-left-60[ 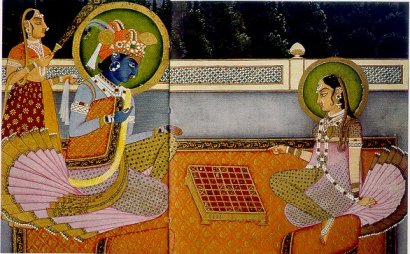 Legend says the inventor of chess (in India) so delighted the raja, he was offered anything he wanted. Inventor said, not much. One grain of rice on one square, 2 on the next, 4 on the next and so on till the board is filled. ] -- .pull-right-40[ 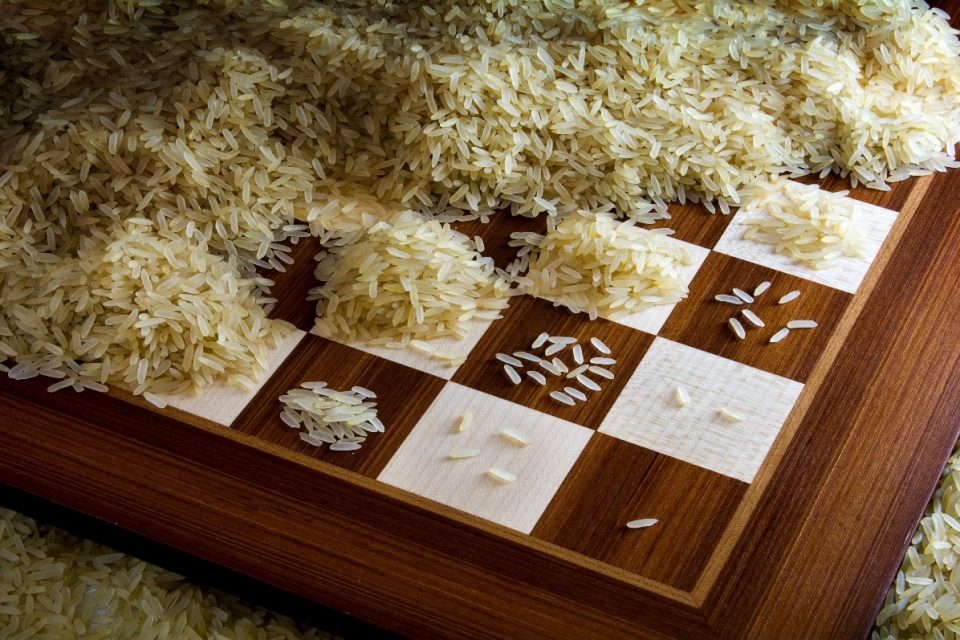 Not only is there not enough rice in India to fill such a chessboard, **there are not enough atoms on earth**. ] --- ## Note what the log-scale does 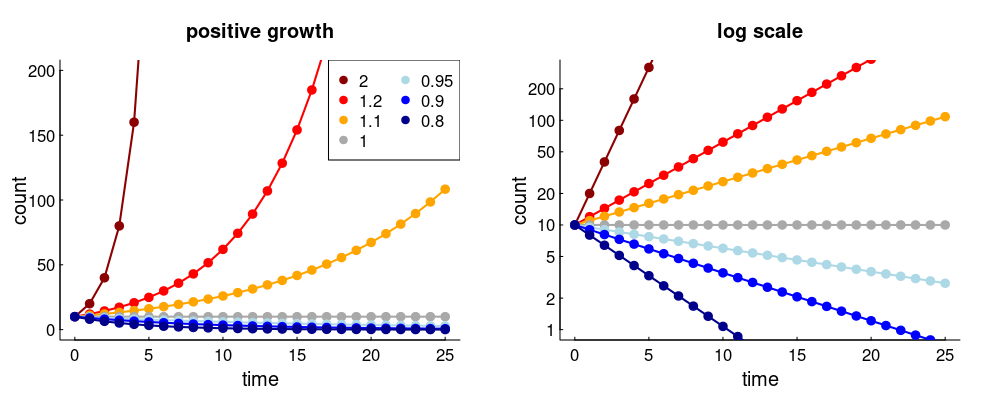<!-- --> --- ## Estimating some rates ... discrete .pull-left-30[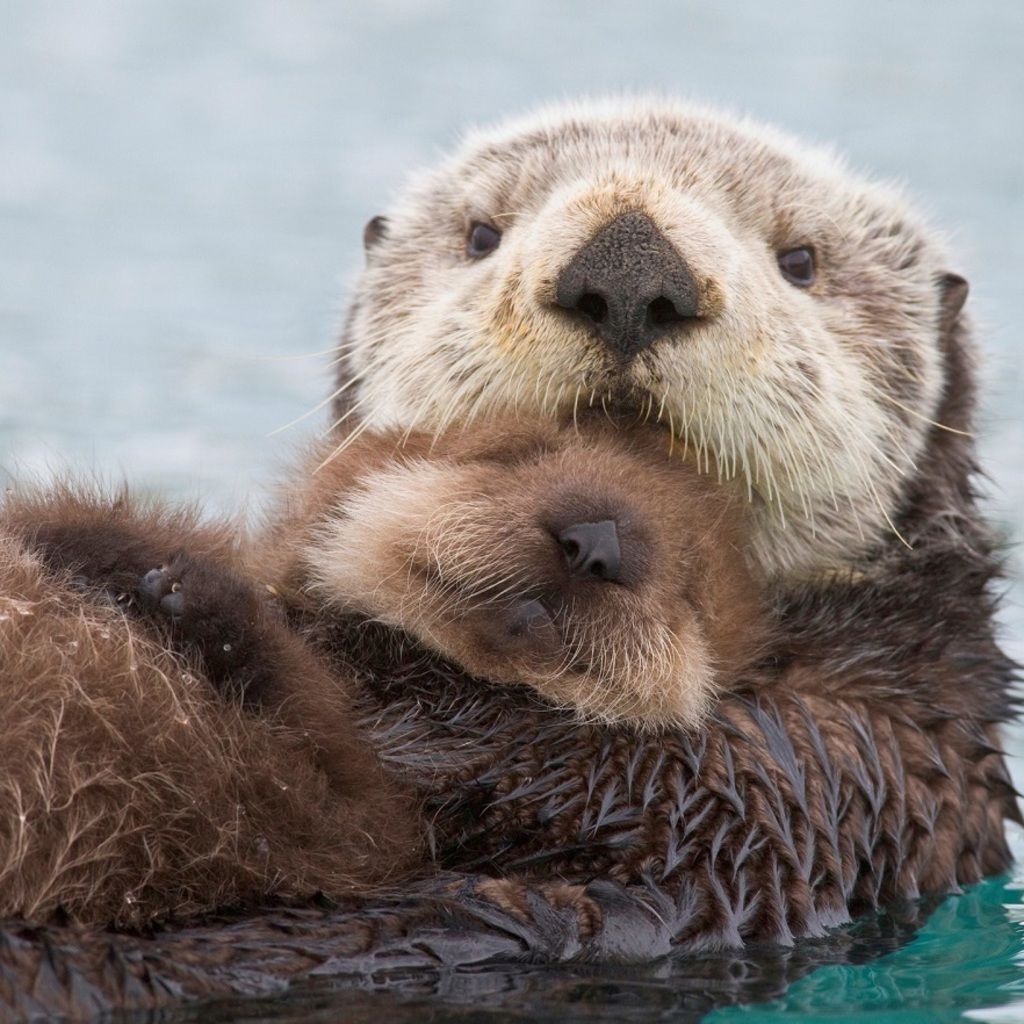] .pull-right-70[ The amazing thing is, if you have an equation "solved", you only need 2 points on the curve to compute. Let's use the discrete equation: `$$N_{t+y} = \lambda^y N_t$$` `$$1000 = 60 \times \lambda^{40}$$` `$$16.7 = \lambda^{40}$$` `$$2.81 = 40 \times \log (\lambda)$$` `$$0.07025 = \log (\lambda)$$` `$$\lambda = \exp(0.07025) = 1.0728$$` i.e. population increase about `$$7.28\%/year$$`. ] --- ## Washington sea otter fit to data (7.025% discrete growth) .pull-left-70[ 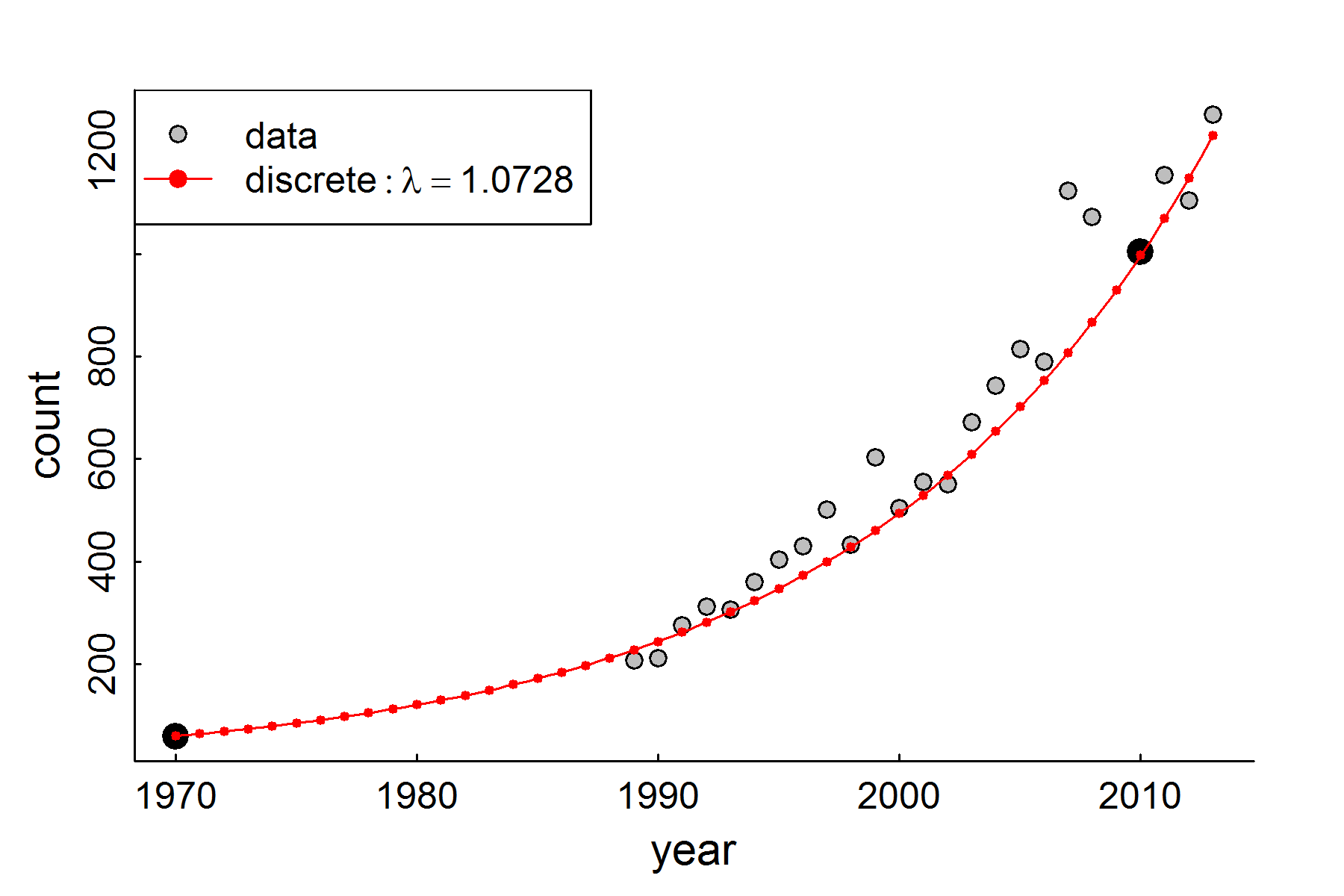 ] .pull-right-30[ ### This is an EXCELLENT fit but why isn't it *perfect*? .darkgreen[What are some potential sources of **variation**?] ] --- ## Sea otter references - J. A. Estes, J. F. Palmisano. 1974. Sea otters: Their role in structuring nearshore communities. Science 185, 1058–1060. - Loshbaugh S. 2021. Sea Otters and the Maritime Fur Trade. In: Davis R.W., Pagano A.M. (eds) *Ethology and Behavioral Ecology of Sea Otters and Polar Bears. Ethology and Behavioral Ecology of Marine Mammals.* - Gilkinson, A.K., Pearson, H.C., Weltz, F. and Davis, R.W., 2007. Photo‐identification of sea otters using nose scars. *The Journal of Wildlife Management*, 71(6), pp.2045-2051. - Veltre, D.W. “Unangax̂: Coastal People of Far Southwestern Alaska”